79. Automated Deep Learning Practice#
79.1. Introduction#
Previously, we learned about the deep learning framework TensorFlow, and its high-level interface Keras is very popular for its good usability. In this experiment, we will learn about the automated deep learning framework Auto-Keras maintained by the Keras team and use it to complete basic applications.
79.2. Key Points#
Introduction to Auto-Keras
Image Classification Tasks
Text Classification Tasks
Visualization of the Optimal Model
-
Analysis of the Advantages and Disadvantages of AutoML
79.3. Introduction to Auto-Keras#
In this experiment, we choose the Auto-Keras framework to introduce the application of automated deep learning. Auto-Keras is a deep learning framework developed by DATA Lab and maintained by the official Keras team. Currently, Auto-Keras should be one of the ideal choices for automated deep learning applications.
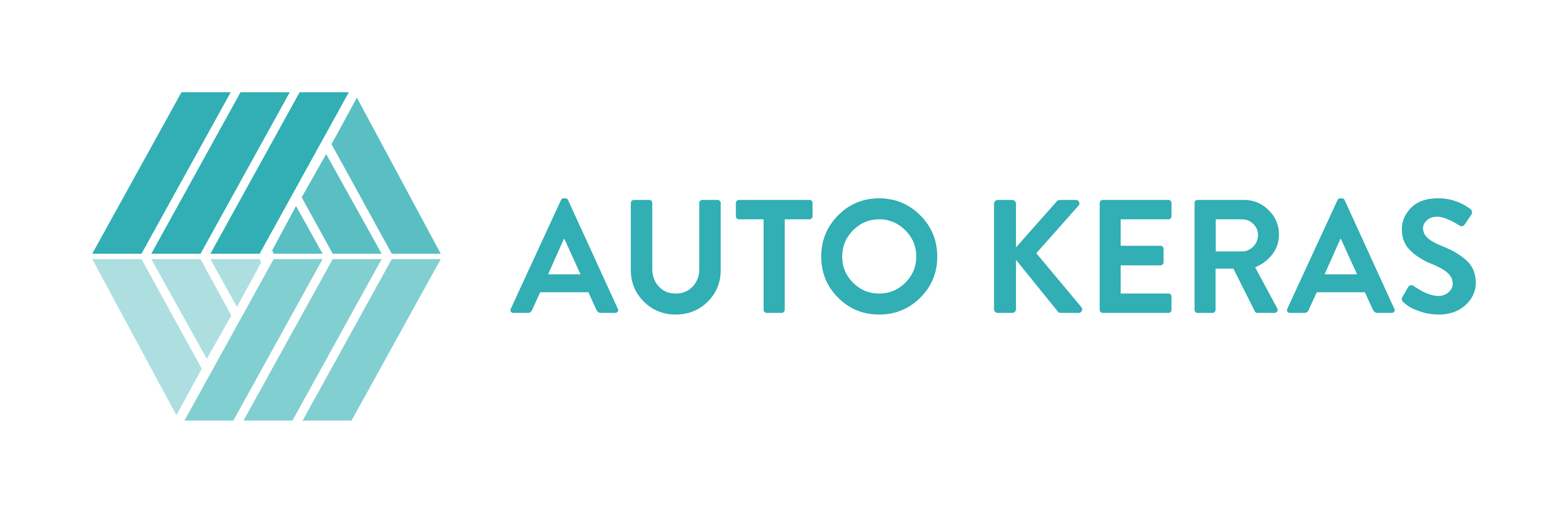
The structure of deep neural networks is the core of deep learning. The appropriate structure and functional layers determine the efficiency and results of deep learning. In actual production environments, we usually follow some classic network structures and make adjustments based on the experience of machine learning experts. However, this process is extremely difficult for those with limited data science or machine learning backgrounds.
Auto-Keras mainly provides two functions: neural architecture search (NAS) and hyperparameter optimization (HPO). Among them, Auto-Keras uses four common NAS methods:
-
Random search: Explore the search space by randomly adjusting the network structure. Therefore, the actual performance of the generated neural network structure has no impact on subsequent searches.
-
Grid search: Search by manually specifying subsets of the hyperparameter space, i.e., the number of network functional layers and the width of the layers are predefined.
-
Greedy search: Explore the search space in a greedy manner. Here, “greedy” means that the network infrastructure for the next search iteration is generated from the structure that has the best performance on the training/validation set in the current iteration.
-
Bayesian optimization: It is the default search strategy in Auto-Keras currently. For more details, please refer to the paper.
It can be seen from the official Auto-Keras repository that its backend mainly relies on scikit-learn and TensorFlow 2 for development currently.
To install Auto-Keras, you can use the command
pip
install
autokeras
.
79.4. Computer Vision#
Computer vision is the most common application scenario in deep learning, which mainly includes: image classification, image generation, object detection, target tracking, semantic segmentation, instance segmentation, etc. Currently, Auto-Keras can complete image classification, while the related tool classes for image segmentation are still under construction.
79.4.1. Image Classification#
For example, the DIGITS and MNIST handwritten character
recognition completed in the previous experiments are
actually an image classification task. The class used for
image classification in Auto-Keras is
autokeras.ImageClassifier
.
Among them, Auto-Keras supports image classification tasks in 2 scenarios. They are:
-
The images have been processed into NumPy arrays, such as the DIGITS and MNIST datasets used in the previous experiments.
-
The images are still in their original JPG, PNG, etc. formats and require preprocessing steps.
Next, we try to use Auto-Keras to complete the applications in these two scenarios. For the task where the images have been processed into NumPy arrays, we also choose the MNIST handwritten character classification. Here, we choose to use the data API provided by TensorFlow to load the data and complete the import.
import tensorflow as tf
# 加载数据集
(X_train, y_train), (X_test, y_test) = tf.keras.datasets.mnist.load_data()
# 查看数组形状
X_train.shape, y_train.shape, X_test.shape, y_test.shape
((60000, 28, 28), (60000,), (10000, 28, 28), (10000,))
It can be seen that the training set has 60,000 samples, the test set has 10,000 samples, and the shape of the sample data is grayscale images of \(28 \times 28\).
Next, we import the class for image classification provided by Auto-Keras and complete the model training process. The model class mainly has 4 methods, which are:
-
fit
: Used to train the model by passing in NumPy arrays. -
predict
: Used for the inference process of the model. -
evaluate
: Used for the evaluation process of the model. -
export_model
: Selects the best model from the tested models and returns it.
Let’s try to use this function to recognize handwritten characters in MNIST (the following code needs to be trained twice in total, so please be patient and wait for 5 - 7 minutes):
import autokeras as ak
# 实例化模型,max_trials=1 ,表示尝试的最大的 神经网络模型数
clf = ak.ImageClassifier(max_trials=1)
# epochs 表示每个模型训练的最大世代数
# batch_size 指定每个 batch 的大小
clf.fit(X_train, y_train, batch_size=1000, epochs=1)
print("训练完成")
Trial 1 Complete [00h 00m 24s]
val_loss: 0.2161960005760193
Best val_loss So Far: 0.2161960005760193
Total elapsed time: 00h 00m 24s
60/60 [==============================] - 27s 441ms/step - loss: 0.5438 - accuracy: 0.8388
INFO:tensorflow:Assets written to: ./image_classifier/best_model/assets
训练完成
Since the training time of the model is very long, only 1
model, i.e.,
max_trials=1
, is tried in the above code (in actual practice, you can
adjust the number of models you want to try according to
your own needs).
During the training process, Auto-Keras will train
max_trials
models respectively. During the training process,
AutoKeras will split the training data into two parts, one
for training and one for evaluation. Then, according to
the evaluation results, it will select the best model
structure among these models, and then put all the data
into this structure for training. Therefore, if you need
to output the best model from
max_trials
models, you must train
max_trials
+
1
times.
Now let me use the selected best model to test the test data:
clf.evaluate(X_test, y_test)
313/313 [==============================] - 2s 6ms/step - loss: 0.1587 - accuracy: 0.9566
[0.1587049812078476, 0.95660001039505]
As can be seen from the results, with Auto-Keras, we also let the computer do the work of building neural networks. Although it takes some time to find the best model, we can still train a good model.
Finally, let’s export the model from Auto-Keras to get the familiar TensorFlow model and display it:
model = clf.export_model()
model.summary()
Model: "model"
_________________________________________________________________
Layer (type) Output Shape Param #
=================================================================
input_1 (InputLayer) [(None, 28, 28)] 0
cast_to_float32 (CastToFlo (None, 28, 28) 0
at32)
expand_last_dim (ExpandLas (None, 28, 28, 1) 0
tDim)
normalization (Normalizati (None, 28, 28, 1) 3
on)
conv2d (Conv2D) (None, 26, 26, 32) 320
conv2d_1 (Conv2D) (None, 24, 24, 64) 18496
max_pooling2d (MaxPooling2 (None, 12, 12, 64) 0
D)
dropout (Dropout) (None, 12, 12, 64) 0
flatten (Flatten) (None, 9216) 0
dropout_1 (Dropout) (None, 9216) 0
dense (Dense) (None, 10) 92170
classification_head_1 (Sof (None, 10) 0
tmax)
=================================================================
Total params: 110989 (433.55 KB)
Trainable params: 110986 (433.54 KB)
Non-trainable params: 3 (16.00 Byte)
_________________________________________________________________
As shown in the figure above, it is the final model structure selected by Auto-Keras.
Thus, it can be seen that the use of Auto-Keras is indeed very simple. You don’t need to consider how to build the network, define the loss function, etc. You only need to pass the data into the API.
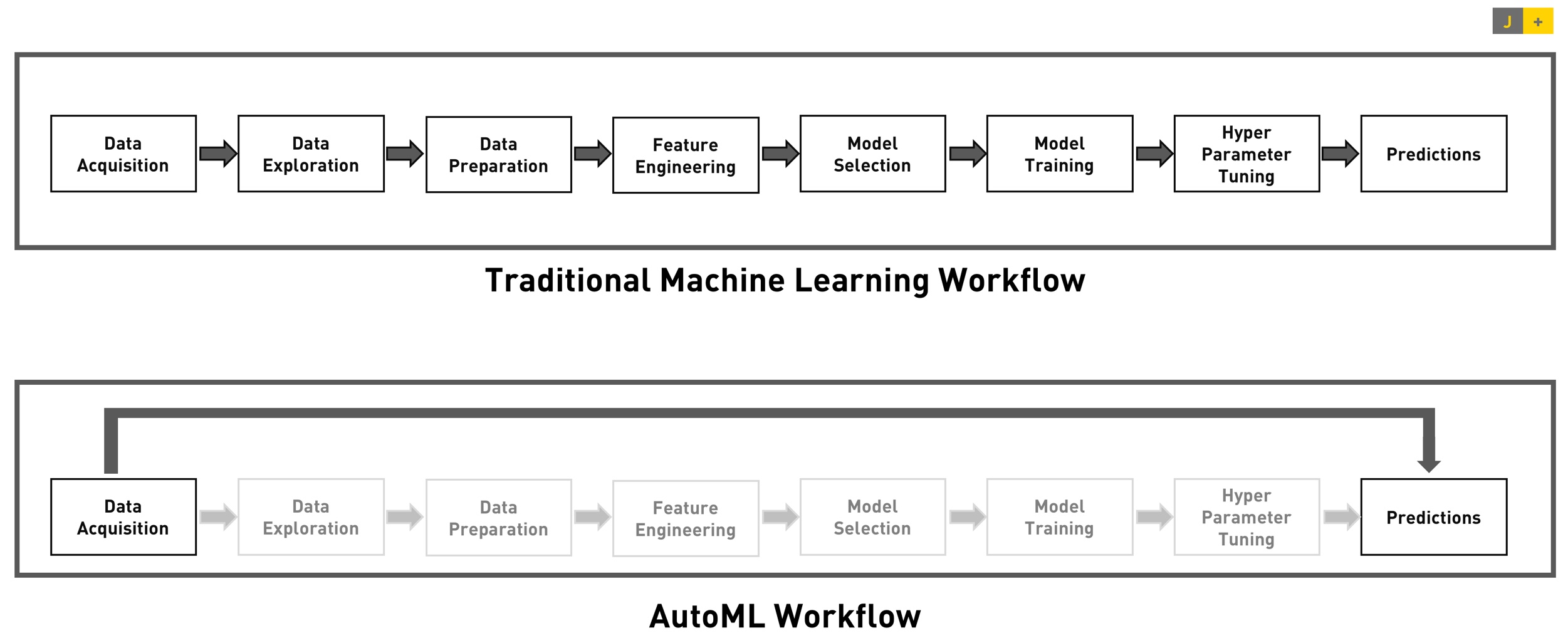
As shown in the figure, traditional machine learning generally requires about 8 steps from data processing to final prediction, while the AutoML process can be simplified to 2 steps. As for data preprocessing, model selection, hyperparameter tuning, etc., all can be left to the framework to complete.
79.5. Natural Language Processing#
In addition to computer vision, natural language processing is also an important application direction of deep learning. Natural language processing mainly studies various theories and methods for effective communication between humans and computers using natural language. Currently, natural language processing has a very rich range of applications, mainly including: information retrieval, speech recognition, machine translation, intelligent question answering, dialogue systems, text classification, sentiment analysis, text generation, automatic summarization, etc.
79.5.1. Text Classification#
The class used to complete text classification in
Auto-Keras is
autokeras.TextClassifier
. Next, we will try to use Auto-Keras to complete the
prediction of text classification for IMDB movie reviews.
The IMDB data is sourced from the famous Internet Movie Database. This dataset consists of movie review data, which is labeled as positive (1) or negative (0).
We can use the interface provided by the official TensorFlow to load the dataset:
import autokeras as ak
import numpy as np
import tensorflow as tf
# 加载 IMDB数据并对它进行处理
max_features = 20000
index_offset = 3
# 加载数据
(x_train, y_train), (x_test, y_test) = tf.keras.datasets.imdb.load_data(
num_words=max_features,
index_from=index_offset)
x_train = x_train
y_train = y_train.reshape(-1, 1)
x_test = x_test
y_test = y_test.reshape(-1, 1)
x_train
array([list([1, 14, 22, 16, 43, 530, 973, 1622, 1385, 65, 458, 4468, 66, 3941, 4, 173, 36, 256, 5, 25, 100, 43, 838, 112, 50, 670, 2, 9, 35, 480, 284, 5, 150, 4, 172, 112, 167, 2, 336, 385, 39, 4, 172, 4536, 1111, 17, 546, 38, 13, 447, 4, 192, 50, 16, 6, 147, 2025, 19, 14, 22, 4, 1920, 4613, 469, 4, 22, 71, 87, 12, 16, 43, 530, 38, 76, 15, 13, 1247, 4, 22, 17, 515, 17, 12, 16, 626, 18, 19193, 5, 62, 386, 12, 8, 316, 8, 106, 5, 4, 2223, 5244, 16, 480, 66, 3785, 33, 4, 130, 12, 16, 38, 619, 5, 25, 124, 51, 36, 135, 48, 25, 1415, 33, 6, 22, 12, 215, 28, 77, 52, 5, 14, 407, 16, 82, 10311, 8, 4, 107, 117, 5952, 15, 256, 4, 2, 7, 3766, 5, 723, 36, 71, 43, 530, 476, 26, 400, 317, 46, 7, 4, 12118, 1029, 13, 104, 88, 4, 381, 15, 297, 98, 32, 2071, 56, 26, 141, 6, 194, 7486, 18, 4, 226, 22, 21, 134, 476, 26, 480, 5, 144, 30, 5535, 18, 51, 36, 28, 224, 92, 25, 104, 4, 226, 65, 16, 38, 1334, 88, 12, 16, 283, 5, 16, 4472, 113, 103, 32, 15, 16, 5345, 19, 178, 32]),
list([1, 194, 1153, 194, 8255, 78, 228, 5, 6, 1463, 4369, 5012, 134, 26, 4, 715, 8, 118, 1634, 14, 394, 20, 13, 119, 954, 189, 102, 5, 207, 110, 3103, 21, 14, 69, 188, 8, 30, 23, 7, 4, 249, 126, 93, 4, 114, 9, 2300, 1523, 5, 647, 4, 116, 9, 35, 8163, 4, 229, 9, 340, 1322, 4, 118, 9, 4, 130, 4901, 19, 4, 1002, 5, 89, 29, 952, 46, 37, 4, 455, 9, 45, 43, 38, 1543, 1905, 398, 4, 1649, 26, 6853, 5, 163, 11, 3215, 10156, 4, 1153, 9, 194, 775, 7, 8255, 11596, 349, 2637, 148, 605, 15358, 8003, 15, 123, 125, 68, 2, 6853, 15, 349, 165, 4362, 98, 5, 4, 228, 9, 43, 2, 1157, 15, 299, 120, 5, 120, 174, 11, 220, 175, 136, 50, 9, 4373, 228, 8255, 5, 2, 656, 245, 2350, 5, 4, 9837, 131, 152, 491, 18, 2, 32, 7464, 1212, 14, 9, 6, 371, 78, 22, 625, 64, 1382, 9, 8, 168, 145, 23, 4, 1690, 15, 16, 4, 1355, 5, 28, 6, 52, 154, 462, 33, 89, 78, 285, 16, 145, 95]),
list([1, 14, 47, 8, 30, 31, 7, 4, 249, 108, 7, 4, 5974, 54, 61, 369, 13, 71, 149, 14, 22, 112, 4, 2401, 311, 12, 16, 3711, 33, 75, 43, 1829, 296, 4, 86, 320, 35, 534, 19, 263, 4821, 1301, 4, 1873, 33, 89, 78, 12, 66, 16, 4, 360, 7, 4, 58, 316, 334, 11, 4, 1716, 43, 645, 662, 8, 257, 85, 1200, 42, 1228, 2578, 83, 68, 3912, 15, 36, 165, 1539, 278, 36, 69, 2, 780, 8, 106, 14, 6905, 1338, 18, 6, 22, 12, 215, 28, 610, 40, 6, 87, 326, 23, 2300, 21, 23, 22, 12, 272, 40, 57, 31, 11, 4, 22, 47, 6, 2307, 51, 9, 170, 23, 595, 116, 595, 1352, 13, 191, 79, 638, 89, 2, 14, 9, 8, 106, 607, 624, 35, 534, 6, 227, 7, 129, 113]),
...,
list([1, 11, 6, 230, 245, 6401, 9, 6, 1225, 446, 2, 45, 2174, 84, 8322, 4007, 21, 4, 912, 84, 14532, 325, 725, 134, 15271, 1715, 84, 5, 36, 28, 57, 1099, 21, 8, 140, 8, 703, 5, 11656, 84, 56, 18, 1644, 14, 9, 31, 7, 4, 9406, 1209, 2295, 2, 1008, 18, 6, 20, 207, 110, 563, 12, 8, 2901, 17793, 8, 97, 6, 20, 53, 4767, 74, 4, 460, 364, 1273, 29, 270, 11, 960, 108, 45, 40, 29, 2961, 395, 11, 6, 4065, 500, 7, 14492, 89, 364, 70, 29, 140, 4, 64, 4780, 11, 4, 2678, 26, 178, 4, 529, 443, 17793, 5, 27, 710, 117, 2, 8123, 165, 47, 84, 37, 131, 818, 14, 595, 10, 10, 61, 1242, 1209, 10, 10, 288, 2260, 1702, 34, 2901, 17793, 4, 65, 496, 4, 231, 7, 790, 5, 6, 320, 234, 2766, 234, 1119, 1574, 7, 496, 4, 139, 929, 2901, 17793, 7750, 5, 4241, 18, 4, 8497, 13164, 250, 11, 1818, 7561, 4, 4217, 5408, 747, 1115, 372, 1890, 1006, 541, 9303, 7, 4, 59, 11027, 4, 3586, 2]),
list([1, 1446, 7079, 69, 72, 3305, 13, 610, 930, 8, 12, 582, 23, 5, 16, 484, 685, 54, 349, 11, 4120, 2959, 45, 58, 1466, 13, 197, 12, 16, 43, 23, 2, 5, 62, 30, 145, 402, 11, 4131, 51, 575, 32, 61, 369, 71, 66, 770, 12, 1054, 75, 100, 2198, 8, 4, 105, 37, 69, 147, 712, 75, 3543, 44, 257, 390, 5, 69, 263, 514, 105, 50, 286, 1814, 23, 4, 123, 13, 161, 40, 5, 421, 4, 116, 16, 897, 13, 2, 40, 319, 5872, 112, 6700, 11, 4803, 121, 25, 70, 3468, 4, 719, 3798, 13, 18, 31, 62, 40, 8, 7200, 4, 2, 7, 14, 123, 5, 942, 25, 8, 721, 12, 145, 5, 202, 12, 160, 580, 202, 12, 6, 52, 58, 11418, 92, 401, 728, 12, 39, 14, 251, 8, 15, 251, 5, 2, 12, 38, 84, 80, 124, 12, 9, 23]),
list([1, 17, 6, 194, 337, 7, 4, 204, 22, 45, 254, 8, 106, 14, 123, 4, 12815, 270, 14437, 5, 16923, 12255, 732, 2098, 101, 405, 39, 14, 1034, 4, 1310, 9, 115, 50, 305, 12, 47, 4, 168, 5, 235, 7, 38, 111, 699, 102, 7, 4, 4039, 9245, 9, 24, 6, 78, 1099, 17, 2345, 16553, 21, 27, 9685, 6139, 5, 2, 1603, 92, 1183, 4, 1310, 7, 4, 204, 42, 97, 90, 35, 221, 109, 29, 127, 27, 118, 8, 97, 12, 157, 21, 6789, 2, 9, 6, 66, 78, 1099, 4, 631, 1191, 5, 2642, 272, 191, 1070, 6, 7585, 8, 2197, 2, 10755, 544, 5, 383, 1271, 848, 1468, 12183, 497, 16876, 8, 1597, 8778, 19280, 21, 60, 27, 239, 9, 43, 8368, 209, 405, 10, 10, 12, 764, 40, 4, 248, 20, 12, 16, 5, 174, 1791, 72, 7, 51, 6, 1739, 22, 4, 204, 131, 9])],
dtype=object)
As can be seen from the results, the data text has been converted into word vectors at this time. However, since the text classifier of Autokeras receives real text, we still need to convert the data back to the original real text. Therefore, we need a correspondence table between text words and numbers, as follows:
# 加载词向量表
word_to_id = tf.keras.datasets.imdb.get_word_index()
word_to_id = {k: (v + index_offset) for k, v in word_to_id.items()}
word_to_id["<PAD>"] = 0
word_to_id["<START>"] = 1
word_to_id["<UNK>"] = 2
id_to_word = {value: key for key, value in word_to_id.items()}
id_to_word
{34704: 'fawn',
52009: 'tsukino',
52010: 'nunnery',
16819: 'sonja',
63954: 'vani',
1411: 'woods',
16118: 'spiders',
2348: 'hanging',
2292: 'woody',
52011: 'trawling',
52012: "hold's",
11310: 'comically',
40833: 'localized',
30571: 'disobeying',
52013: "'royale",
40834: "harpo's",
52014: 'canet',
19316: 'aileen',
52015: 'acurately',
52016: "diplomat's",
25245: 'rickman',
6749: 'arranged',
52017: 'rumbustious',
52018: 'familiarness',
52019: "spider'",
68807: 'hahahah',
52020: "wood'",
40836: 'transvestism',
34705: "hangin'",
2341: 'bringing',
40837: 'seamier',
34706: 'wooded',
52021: 'bravora',
16820: 'grueling',
1639: 'wooden',
16821: 'wednesday',
52022: "'prix",
34707: 'altagracia',
52023: 'circuitry',
11588: 'crotch',
57769: 'busybody',
52024: "tart'n'tangy",
14132: 'burgade',
52026: 'thrace',
11041: "tom's",
52028: 'snuggles',
29117: 'francesco',
52030: 'complainers',
52128: 'templarios',
40838: '272',
52031: '273',
52133: 'zaniacs',
34709: '275',
27634: 'consenting',
40839: 'snuggled',
15495: 'inanimate',
52033: 'uality',
11929: 'bronte',
4013: 'errors',
3233: 'dialogs',
52034: "yomada's",
34710: "madman's",
30588: 'dialoge',
52036: 'usenet',
40840: 'videodrome',
26341: "kid'",
52037: 'pawed',
30572: "'girlfriend'",
52038: "'pleasure",
52039: "'reloaded'",
40842: "kazakos'",
52040: 'rocque',
52041: 'mailings',
11930: 'brainwashed',
16822: 'mcanally',
52042: "tom''",
25246: 'kurupt',
21908: 'affiliated',
52043: 'babaganoosh',
40843: "noe's",
40844: 'quart',
362: 'kids',
5037: 'uplifting',
7096: 'controversy',
21909: 'kida',
23382: 'kidd',
52044: "error'",
52045: 'neurologist',
18513: 'spotty',
30573: 'cobblers',
9881: 'projection',
40845: 'fastforwarding',
52046: 'sters',
52047: "eggar's",
52048: 'etherything',
40846: 'gateshead',
34711: 'airball',
25247: 'unsinkable',
7183: 'stern',
52049: "cervi's",
40847: 'dnd',
11589: 'dna',
20601: 'insecurity',
52050: "'reboot'",
11040: 'trelkovsky',
52051: 'jaekel',
52052: 'sidebars',
52053: "sforza's",
17636: 'distortions',
52054: 'mutinies',
30605: 'sermons',
40849: '7ft',
52055: 'boobage',
52056: "o'bannon's",
23383: 'populations',
52057: 'chulak',
27636: 'mesmerize',
52058: 'quinnell',
10310: 'yahoo',
52060: 'meteorologist',
42580: 'beswick',
15496: 'boorman',
40850: 'voicework',
52061: "ster'",
22925: 'blustering',
52062: 'hj',
27637: 'intake',
5624: 'morally',
40852: 'jumbling',
52063: 'bowersock',
52064: "'porky's'",
16824: 'gershon',
40853: 'ludicrosity',
52065: 'coprophilia',
40854: 'expressively',
19503: "india's",
34713: "post's",
52066: 'wana',
5286: 'wang',
30574: 'wand',
25248: 'wane',
52324: 'edgeways',
34714: 'titanium',
40855: 'pinta',
181: 'want',
30575: 'pinto',
52068: 'whoopdedoodles',
21911: 'tchaikovsky',
2106: 'travel',
52069: "'victory'",
11931: 'copious',
22436: 'gouge',
52070: "chapters'",
6705: 'barbra',
30576: 'uselessness',
52071: "wan'",
27638: 'assimilated',
16119: 'petiot',
52072: 'most\x85and',
3933: 'dinosaurs',
355: 'wrong',
52073: 'seda',
52074: 'stollen',
34715: 'sentencing',
40856: 'ouroboros',
40857: 'assimilates',
40858: 'colorfully',
27639: 'glenne',
52075: 'dongen',
4763: 'subplots',
52076: 'kiloton',
23384: 'chandon',
34716: "effect'",
27640: 'snugly',
40859: 'kuei',
9095: 'welcomed',
30074: 'dishonor',
52078: 'concurrence',
23385: 'stoicism',
14899: "guys'",
52080: "beroemd'",
6706: 'butcher',
40860: "melfi's",
30626: 'aargh',
20602: 'playhouse',
11311: 'wickedly',
1183: 'fit',
52081: 'labratory',
40862: 'lifeline',
1930: 'screaming',
4290: 'fix',
52082: 'cineliterate',
52083: 'fic',
52084: 'fia',
34717: 'fig',
52085: 'fmvs',
52086: 'fie',
52087: 'reentered',
30577: 'fin',
52088: 'doctresses',
52089: 'fil',
12609: 'zucker',
31934: 'ached',
52091: 'counsil',
52092: 'paterfamilias',
13888: 'songwriter',
34718: 'shivam',
9657: 'hurting',
302: 'effects',
52093: 'slauther',
52094: "'flame'",
52095: 'sommerset',
52096: 'interwhined',
27641: 'whacking',
52097: 'bartok',
8778: 'barton',
21912: 'frewer',
52098: "fi'",
6195: 'ingrid',
30578: 'stribor',
52099: 'approporiately',
52100: 'wobblyhand',
52101: 'tantalisingly',
52102: 'ankylosaurus',
17637: 'parasites',
52103: 'childen',
52104: "jenkins'",
52105: 'metafiction',
17638: 'golem',
40863: 'indiscretion',
23386: "reeves'",
57784: "inamorata's",
52107: 'brittannica',
7919: 'adapt',
30579: "russo's",
48249: 'guitarists',
10556: 'abbott',
40864: 'abbots',
17652: 'lanisha',
40866: 'magickal',
52108: 'mattter',
52109: "'willy",
34719: 'pumpkins',
52110: 'stuntpeople',
30580: 'estimate',
40867: 'ugghhh',
11312: 'gameplay',
52111: "wern't",
40868: "n'sync",
16120: 'sickeningly',
40869: 'chiara',
4014: 'disturbed',
40870: 'portmanteau',
52112: 'ineffectively',
82146: "duchonvey's",
37522: "nasty'",
1288: 'purpose',
52115: 'lazers',
28108: 'lightened',
52116: 'kaliganj',
52117: 'popularism',
18514: "damme's",
30581: 'stylistics',
52118: 'mindgaming',
46452: 'spoilerish',
52120: "'corny'",
34721: 'boerner',
6795: 'olds',
52121: 'bakelite',
27642: 'renovated',
27643: 'forrester',
52122: "lumiere's",
52027: 'gaskets',
887: 'needed',
34722: 'smight',
1300: 'master',
25908: "edie's",
40871: 'seeber',
52123: 'hiya',
52124: 'fuzziness',
14900: 'genesis',
12610: 'rewards',
30582: 'enthrall',
40872: "'about",
52125: "recollection's",
11042: 'mutilated',
52126: 'fatherlands',
52127: "fischer's",
5402: 'positively',
34708: '270',
34723: 'ahmed',
9839: 'zatoichi',
13889: 'bannister',
52130: 'anniversaries',
30583: "helm's",
52131: "'work'",
34724: 'exclaimed',
52132: "'unfunny'",
52032: '274',
547: 'feeling',
52134: "wanda's",
33269: 'dolan',
52136: '278',
52137: 'peacoat',
40873: 'brawny',
40874: 'mishra',
40875: 'worlders',
52138: 'protags',
52139: 'skullcap',
57599: 'dastagir',
5625: 'affairs',
7802: 'wholesome',
52140: 'hymen',
25249: 'paramedics',
52141: 'unpersons',
52142: 'heavyarms',
52143: 'affaire',
52144: 'coulisses',
40876: 'hymer',
52145: 'kremlin',
30584: 'shipments',
52146: 'pixilated',
30585: "'00s",
18515: 'diminishing',
1360: 'cinematic',
14901: 'resonates',
40877: 'simplify',
40878: "nature'",
40879: 'temptresses',
16825: 'reverence',
19505: 'resonated',
34725: 'dailey',
52147: '2\x85',
27644: 'treize',
52148: 'majo',
21913: 'kiya',
52149: 'woolnough',
39800: 'thanatos',
35734: 'sandoval',
40882: 'dorama',
52150: "o'shaughnessy",
4991: 'tech',
32021: 'fugitives',
30586: 'teck',
76128: "'e'",
40884: 'doesn’t',
52152: 'purged',
660: 'saying',
41098: "martians'",
23421: 'norliss',
27645: 'dickey',
52155: 'dicker',
52156: "'sependipity",
8425: 'padded',
57795: 'ordell',
40885: "sturges'",
52157: 'independentcritics',
5748: 'tempted',
34727: "atkinson's",
25250: 'hounded',
52158: 'apace',
15497: 'clicked',
30587: "'humor'",
17180: "martino's",
52159: "'supporting",
52035: 'warmongering',
34728: "zemeckis's",
21914: 'lube',
52160: 'shocky',
7479: 'plate',
40886: 'plata',
40887: 'sturgess',
40888: "nerds'",
20603: 'plato',
34729: 'plath',
40889: 'platt',
52162: 'mcnab',
27646: 'clumsiness',
3902: 'altogether',
42587: 'massacring',
52163: 'bicenntinial',
40890: 'skaal',
14363: 'droning',
8779: 'lds',
21915: 'jaguar',
34730: "cale's",
1780: 'nicely',
4591: 'mummy',
18516: "lot's",
10089: 'patch',
50205: 'kerkhof',
52164: "leader's",
27647: "'movie",
52165: 'uncomfirmed',
40891: 'heirloom',
47363: 'wrangle',
52166: 'emotion\x85',
52167: "'stargate'",
40892: 'pinoy',
40893: 'conchatta',
41131: 'broeke',
40894: 'advisedly',
17639: "barker's",
52169: 'descours',
775: 'lots',
9262: 'lotr',
9882: 'irs',
52170: 'lott',
40895: 'xvi',
34731: 'irk',
52171: 'irl',
6890: 'ira',
21916: 'belzer',
52172: 'irc',
27648: 'ire',
40896: 'requisites',
7696: 'discipline',
52964: 'lyoko',
11313: 'extend',
876: 'nature',
52173: "'dickie'",
40897: 'optimist',
30589: 'lapping',
3903: 'superficial',
52174: 'vestment',
2826: 'extent',
52175: 'tendons',
52176: "heller's",
52177: 'quagmires',
52178: 'miyako',
20604: 'moocow',
52179: "coles'",
40898: 'lookit',
52180: 'ravenously',
40899: 'levitating',
52181: 'perfunctorily',
30590: 'lookin',
40901: "lot'",
52182: 'lookie',
34873: 'fearlessly',
52184: 'libyan',
40902: 'fondles',
35717: 'gopher',
40904: 'wearying',
52185: "nz's",
27649: 'minuses',
52186: 'puposelessly',
52187: 'shandling',
31271: 'decapitates',
11932: 'humming',
40905: "'nother",
21917: 'smackdown',
30591: 'underdone',
40906: 'frf',
52188: 'triviality',
25251: 'fro',
8780: 'bothers',
52189: "'kensington",
76: 'much',
34733: 'muco',
22618: 'wiseguy',
27651: "richie's",
40907: 'tonino',
52190: 'unleavened',
11590: 'fry',
40908: "'tv'",
40909: 'toning',
14364: 'obese',
30592: 'sensationalized',
40910: 'spiv',
6262: 'spit',
7367: 'arkin',
21918: 'charleton',
16826: 'jeon',
21919: 'boardroom',
4992: 'doubts',
3087: 'spin',
53086: 'hepo',
27652: 'wildcat',
10587: 'venoms',
52194: 'misconstrues',
18517: 'mesmerising',
40911: 'misconstrued',
52195: 'rescinds',
52196: 'prostrate',
40912: 'majid',
16482: 'climbed',
34734: 'canoeing',
52198: 'majin',
57807: 'animie',
40913: 'sylke',
14902: 'conditioned',
40914: 'waddell',
52199: '3\x85',
41191: 'hyperdrive',
34735: 'conditioner',
53156: 'bricklayer',
2579: 'hong',
52201: 'memoriam',
30595: 'inventively',
25252: "levant's",
20641: 'portobello',
52203: 'remand',
19507: 'mummified',
27653: 'honk',
19508: 'spews',
40915: 'visitations',
52204: 'mummifies',
25253: 'cavanaugh',
23388: 'zeon',
40916: "jungle's",
34736: 'viertel',
27654: 'frenchmen',
52205: 'torpedoes',
52206: 'schlessinger',
34737: 'torpedoed',
69879: 'blister',
52207: 'cinefest',
34738: 'furlough',
52208: 'mainsequence',
40917: 'mentors',
9097: 'academic',
20605: 'stillness',
40918: 'academia',
52209: 'lonelier',
52210: 'nibby',
52211: "losers'",
40919: 'cineastes',
4452: 'corporate',
40920: 'massaging',
30596: 'bellow',
19509: 'absurdities',
53244: 'expetations',
40921: 'nyfiken',
75641: 'mehras',
52212: 'lasse',
52213: 'visability',
33949: 'militarily',
52214: "elder'",
19026: 'gainsbourg',
20606: 'hah',
13423: 'hai',
34739: 'haj',
25254: 'hak',
4314: 'hal',
4895: 'ham',
53262: 'duffer',
52216: 'haa',
69: 'had',
11933: 'advancement',
16828: 'hag',
25255: "hand'",
13424: 'hay',
20607: 'mcnamara',
52217: "mozart's",
30734: 'duffel',
30597: 'haq',
13890: 'har',
47: 'has',
2404: 'hat',
40922: 'hav',
30598: 'haw',
52218: 'figtings',
15498: 'elders',
52219: 'underpanted',
52220: 'pninson',
27655: 'unequivocally',
23676: "barbara's",
52222: "bello'",
13000: 'indicative',
40923: 'yawnfest',
52223: 'hexploitation',
52224: "loder's",
27656: 'sleuthing',
32625: "justin's",
52225: "'ball",
52226: "'summer",
34938: "'demons'",
52228: "mormon's",
34740: "laughton's",
52229: 'debell',
39727: 'shipyard',
30600: 'unabashedly',
40404: 'disks',
2293: 'crowd',
10090: 'crowe',
56437: "vancouver's",
34741: 'mosques',
6630: 'crown',
52230: 'culpas',
27657: 'crows',
53347: 'surrell',
52232: 'flowless',
52233: 'sheirk',
40926: "'three",
52234: "peterson'",
52235: 'ooverall',
40927: 'perchance',
1324: 'bottom',
53366: 'chabert',
52236: 'sneha',
13891: 'inhuman',
52237: 'ichii',
52238: 'ursla',
30601: 'completly',
40928: 'moviedom',
52239: 'raddick',
51998: 'brundage',
40929: 'brigades',
1184: 'starring',
52240: "'goal'",
52241: 'caskets',
52242: 'willcock',
52243: "threesome's",
52244: "mosque'",
52245: "cover's",
17640: 'spaceships',
40930: 'anomalous',
27658: 'ptsd',
52246: 'shirdan',
21965: 'obscenity',
30602: 'lemmings',
30603: 'duccio',
52247: "levene's",
52248: "'gorby'",
25258: "teenager's",
5343: 'marshall',
9098: 'honeymoon',
3234: 'shoots',
12261: 'despised',
52249: 'okabasho',
8292: 'fabric',
18518: 'cannavale',
3540: 'raped',
52250: "tutt's",
17641: 'grasping',
18519: 'despises',
40931: "thief's",
8929: 'rapes',
52251: 'raper',
27659: "eyre'",
52252: 'walchek',
23389: "elmo's",
40932: 'perfumes',
21921: 'spurting',
52253: "exposition'\x85",
52254: 'denoting',
34743: 'thesaurus',
40933: "shoot'",
49762: 'bonejack',
52256: 'simpsonian',
30604: 'hebetude',
34744: "hallow's",
52257: 'desperation\x85',
34745: 'incinerator',
10311: 'congratulations',
52258: 'humbled',
5927: "else's",
40848: 'trelkovski',
52259: "rape'",
59389: "'chapters'",
52260: '1600s',
7256: 'martian',
25259: 'nicest',
52262: 'eyred',
9460: 'passenger',
6044: 'disgrace',
52263: 'moderne',
5123: 'barrymore',
52264: 'yankovich',
40934: 'moderns',
52265: 'studliest',
52266: 'bedsheet',
14903: 'decapitation',
52267: 'slurring',
52268: "'nunsploitation'",
34746: "'character'",
9883: 'cambodia',
52269: 'rebelious',
27660: 'pasadena',
40935: 'crowne',
52270: "'bedchamber",
52271: 'conjectural',
52272: 'appologize',
52273: 'halfassing',
57819: 'paycheque',
20609: 'palms',
52274: "'islands",
40936: 'hawked',
21922: 'palme',
40937: 'conservatively',
64010: 'larp',
5561: 'palma',
21923: 'smelling',
13001: 'aragorn',
52275: 'hawker',
52276: 'hawkes',
3978: 'explosions',
8062: 'loren',
52277: "pyle's",
6707: 'shootout',
18520: "mike's",
52278: "driscoll's",
40938: 'cogsworth',
52279: "britian's",
34747: 'childs',
52280: "portrait's",
3629: 'chain',
2500: 'whoever',
52281: 'puttered',
52282: 'childe',
52283: 'maywether',
3039: 'chair',
52284: "rance's",
34748: 'machu',
4520: 'ballet',
34749: 'grapples',
76155: 'summerize',
30606: 'freelance',
52286: "andrea's",
52287: '\x91very',
45882: 'coolidge',
18521: 'mache',
52288: 'balled',
40940: 'grappled',
18522: 'macha',
21924: 'underlining',
5626: 'macho',
19510: 'oversight',
25260: 'machi',
11314: 'verbally',
21925: 'tenacious',
40941: 'windshields',
18560: 'paychecks',
3399: 'jerk',
11934: "good'",
34751: 'prancer',
21926: 'prances',
52289: 'olympus',
21927: 'lark',
10788: 'embark',
7368: 'gloomy',
52290: 'jehaan',
52291: 'turaqui',
20610: "child'",
2897: 'locked',
52292: 'pranced',
2591: 'exact',
52293: 'unattuned',
786: 'minute',
16121: 'skewed',
40943: 'hodgins',
34752: 'skewer',
52294: 'think\x85',
38768: 'rosenstein',
52295: 'helmit',
34753: 'wrestlemanias',
16829: 'hindered',
30607: "martha's",
52296: 'cheree',
52297: "pluckin'",
40944: 'ogles',
11935: 'heavyweight',
82193: 'aada',
11315: 'chopping',
61537: 'strongboy',
41345: 'hegemonic',
40945: 'adorns',
41349: 'xxth',
34754: 'nobuhiro',
52301: 'capitães',
52302: 'kavogianni',
13425: 'antwerp',
6541: 'celebrated',
52303: 'roarke',
40946: 'baggins',
31273: 'cheeseburgers',
52304: 'matras',
52305: "nineties'",
52306: "'craig'",
13002: 'celebrates',
3386: 'unintentionally',
14365: 'drafted',
52307: 'climby',
52308: '303',
18523: 'oldies',
9099: 'climbs',
9658: 'honour',
34755: 'plucking',
30077: '305',
5517: 'address',
40947: 'menjou',
42595: "'freak'",
19511: 'dwindling',
9461: 'benson',
52310: 'white’s',
40948: 'shamelessness',
21928: 'impacted',
52311: 'upatz',
3843: 'cusack',
37570: "flavia's",
52312: 'effette',
34756: 'influx',
52313: 'boooooooo',
52314: 'dimitrova',
13426: 'houseman',
25262: 'bigas',
52315: 'boylen',
52316: 'phillipenes',
40949: 'fakery',
27661: "grandpa's",
27662: 'darnell',
19512: 'undergone',
52318: 'handbags',
21929: 'perished',
37781: 'pooped',
27663: 'vigour',
3630: 'opposed',
52319: 'etude',
11802: "caine's",
52320: 'doozers',
34757: 'photojournals',
52321: 'perishes',
34758: 'constrains',
40951: 'migenes',
30608: 'consoled',
16830: 'alastair',
52322: 'wvs',
52323: 'ooooooh',
34759: 'approving',
40952: 'consoles',
52067: 'disparagement',
52325: 'futureistic',
52326: 'rebounding',
52327: "'date",
52328: 'gregoire',
21930: 'rutherford',
34760: 'americanised',
82199: 'novikov',
1045: 'following',
34761: 'munroe',
52329: "morita'",
52330: 'christenssen',
23109: 'oatmeal',
25263: 'fossey',
40953: 'livered',
13003: 'listens',
76167: "'marci",
52333: "otis's",
23390: 'thanking',
16022: 'maude',
34762: 'extensions',
52335: 'ameteurish',
52336: "commender's",
27664: 'agricultural',
4521: 'convincingly',
17642: 'fueled',
54017: 'mahattan',
40955: "paris's",
52339: 'vulkan',
52340: 'stapes',
52341: 'odysessy',
12262: 'harmon',
4255: 'surfing',
23497: 'halloran',
49583: 'unbelieveably',
52342: "'offed'",
30610: 'quadrant',
19513: 'inhabiting',
34763: 'nebbish',
40956: 'forebears',
34764: 'skirmish',
52343: 'ocassionally',
52344: "'resist",
21931: 'impactful',
52345: 'spicier',
40957: 'touristy',
52346: "'football'",
40958: 'webpage',
52348: 'exurbia',
52349: 'jucier',
14904: 'professors',
34765: 'structuring',
30611: 'jig',
40959: 'overlord',
25264: 'disconnect',
82204: 'sniffle',
40960: 'slimeball',
40961: 'jia',
16831: 'milked',
40962: 'banjoes',
1240: 'jim',
52351: 'workforces',
52352: 'jip',
52353: 'rotweiller',
34766: 'mundaneness',
52354: "'ninja'",
11043: "dead'",
40963: "cipriani's",
20611: 'modestly',
52355: "professor'",
40964: 'shacked',
34767: 'bashful',
23391: 'sorter',
16123: 'overpowering',
18524: 'workmanlike',
27665: 'henpecked',
18525: 'sorted',
52357: "jōb's",
52358: "'always",
34768: "'baptists",
52359: 'dreamcatchers',
52360: "'silence'",
21932: 'hickory',
52361: 'fun\x97yet',
52362: 'breakumentary',
15499: 'didn',
52363: 'didi',
52364: 'pealing',
40965: 'dispite',
25265: "italy's",
21933: 'instability',
6542: 'quarter',
12611: 'quartet',
52365: 'padmé',
52366: "'bleedmedry",
52367: 'pahalniuk',
52368: 'honduras',
10789: 'bursting',
41468: "pablo's",
52370: 'irremediably',
40966: 'presages',
57835: 'bowlegged',
65186: 'dalip',
6263: 'entering',
76175: 'newsradio',
54153: 'presaged',
27666: "giallo's",
40967: 'bouyant',
52371: 'amerterish',
18526: 'rajni',
30613: 'leeves',
34770: 'macauley',
615: 'seriously',
52372: 'sugercoma',
52373: 'grimstead',
52374: "'fairy'",
30614: 'zenda',
52375: "'twins'",
17643: 'realisation',
27667: 'highsmith',
7820: 'raunchy',
40968: 'incentives',
52377: 'flatson',
35100: 'snooker',
16832: 'crazies',
14905: 'crazier',
7097: 'grandma',
52378: 'napunsaktha',
30615: 'workmanship',
52379: 'reisner',
61309: "sanford's",
52380: '\x91doña',
6111: 'modest',
19156: "everything's",
40969: 'hamer',
52382: "couldn't'",
13004: 'quibble',
52383: 'socking',
21934: 'tingler',
52384: 'gutman',
40970: 'lachlan',
52385: 'tableaus',
52386: 'headbanger',
2850: 'spoken',
34771: 'cerebrally',
23493: "'road",
21935: 'tableaux',
40971: "proust's",
40972: 'periodical',
52388: "shoveller's",
25266: 'tamara',
17644: 'affords',
3252: 'concert',
87958: "yara's",
52389: 'someome',
8427: 'lingering',
41514: "abraham's",
34772: 'beesley',
34773: 'cherbourg',
28627: 'kagan',
9100: 'snatch',
9263: "miyazaki's",
25267: 'absorbs',
40973: "koltai's",
64030: 'tingled',
19514: 'crossroads',
16124: 'rehab',
52392: 'falworth',
52393: 'sequals',
...}
As shown above, this correspondence table is like a dictionary, mapping each word to an id, thus converting all strings into numbers.
Next, we need to use this table to convert the training data and test data back to the original text:
x_train = list(map(lambda sentence: ' '.join(id_to_word[i] for i in sentence), x_train))
x_test = list(map(lambda sentence: ' '.join(id_to_word[i] for i in sentence), x_test))
x_train = np.array(x_train, dtype=np.str_)
x_test = np.array(x_test, dtype=np.str_)
print(x_train)
print(x_train.shape) # (25000,)
print(y_train.shape) # (25000, 1)
print(x_train[0][:50]) # <START> this film was just brilliant casting <UNK>
["<START> this film was just brilliant casting location scenery story direction everyone's really suited the part they played and you could just imagine being there robert <UNK> is an amazing actor and now the same being director <UNK> father came from the same scottish island as myself so i loved the fact there was a real connection with this film the witty remarks throughout the film were great it was just brilliant so much that i bought the film as soon as it was released for retail and would recommend it to everyone to watch and the fly fishing was amazing really cried at the end it was so sad and you know what they say if you cry at a film it must have been good and this definitely was also congratulations to the two little boy's that played the <UNK> of norman and paul they were just brilliant children are often left out of the praising list i think because the stars that play them all grown up are such a big profile for the whole film but these children are amazing and should be praised for what they have done don't you think the whole story was so lovely because it was true and was someone's life after all that was shared with us all"
"<START> big hair big boobs bad music and a giant safety pin these are the words to best describe this terrible movie i love cheesy horror movies and i've seen hundreds but this had got to be on of the worst ever made the plot is paper thin and ridiculous the acting is an abomination the script is completely laughable the best is the end showdown with the cop and how he worked out who the killer is it's just so damn terribly written the clothes are sickening and funny in equal measures the hair is big lots of boobs bounce men wear those cut tee shirts that show off their <UNK> sickening that men actually wore them and the music is just <UNK> trash that plays over and over again in almost every scene there is trashy music boobs and <UNK> taking away bodies and the gym still doesn't close for <UNK> all joking aside this is a truly bad film whose only charm is to look back on the disaster that was the 80's and have a good old laugh at how bad everything was back then"
"<START> this has to be one of the worst films of the 1990s when my friends i were watching this film being the target audience it was aimed at we just sat watched the first half an hour with our jaws touching the floor at how bad it really was the rest of the time everyone else in the theatre just started talking to each other leaving or generally crying into their popcorn that they actually paid money they had <UNK> working to watch this feeble excuse for a film it must have looked like a great idea on paper but on film it looks like no one in the film has a clue what is going on crap acting crap costumes i can't get across how <UNK> this is to watch save yourself an hour a bit of your life"
...
"<START> in a far away galaxy is a planet called <UNK> it's native people worship cats but the dog people wage war upon these feline loving people and they have no choice but to go to earth and grind people up for food this is one of the stupidest f k <UNK> ideas for a movie i've seen leave it to ted mikels to make a movie more incompetent than the already low standard he set in previous films it's like he enjoying playing in a celluloid game of limbo how low can he go the only losers in the scenario are us the viewer mr mikels and his silly little <UNK> mustache actually has people who still buy this crap br br my grade f br br dvd extras commentary by ted mikels the story behind the making of 9 and a half minutes 17 minutes 15 seconds of behind the scenes footage ted mikels filmography and trailers for the worm eaters girl in gold boots the doll squad ten violent women featuring nudity blood orgy of the she devils the corpse <UNK>"
"<START> six degrees had me hooked i looked forward to it coming on and was totally disappointed when men in trees replaced it's time spot i thought it was just on <UNK> and would be back early in 2007 what happened all my friends were really surprised it ended we could relate to the characters who had real problems we talked about each episode and had our favorite characters there wasn't anybody on the show i didn't like and felt the acting was superb i <UNK> like seeing programs being taped in cities where you can identify the local areas i for one would like to protest the <UNK> of this show and ask you to bring it back and give it another chance give it a good time slot don't keep moving it from this day to that day and <UNK> it so people will know it is on"
"<START> as a big fan of the original film it's hard to watch this show the garish set decor and harshly lighted sets rob any style from this remake the mood is never there instead it has the look and feel of so many television movies of the seventies crenna is not a bad choice as walter neff but his snappy wardrobe and <UNK> apartment don't fit the mood of the original or make him an interesting character he does his best to make it work but samantha <UNK> is a really bad choice the english accent and california looks can't hold a candle to barbara <UNK> velvet voice and sex appeal lee j cobb tries mightily to fashion barton keyes but even his performance is just gruff without style br br it feels like the tv movie it was and again reminds me of what a remarkable film the original still is"]
(25000,)
(25000, 1)
<START> this film was just brilliant casting locat
As shown in the figure above, we need to evaluate and
predict the above real text. Similar to the
ImageClassifier
method used for image classification, the usage method of
TextClassifier
is as follows (the following code will run for 8 - 10
minutes, please be patient):
# 这里还是只尝试一个模型,1 个 epoch。
# 为了节约资源,这里我们将 max_trials 设置为 1
# 在本地运行时,你可以将 max_trials 设置为 10 左右,进而找到较优的模型
clf = ak.TextClassifier(max_trials=1)
clf.fit(x_train, y_train, epochs=1)
print("训练完成")
Trial 1 Complete [00h 00m 26s]
val_loss: 0.29259392619132996
Best val_loss So Far: 0.29259392619132996
Total elapsed time: 00h 00m 26s
782/782 [==============================] - 32s 40ms/step - loss: 0.4363 - accuracy: 0.7761
INFO:tensorflow:Assets written to: ./text_classifier/best_model/assets
训练完成
{note}
The above results show that a new line will be started every time more than half of a batch has been iterated. Therefore, if you find that the progress bar has stopped moving, you can pull down the display box, as the results may be below.
Similarly, let’s put in the test data and observe the accuracy of the model found:
clf.evaluate(x_test, y_test)
782/782 [==============================] - 10s 13ms/step - loss: 0.2807 - accuracy: 0.8835
[0.28066298365592957, 0.8834800124168396]
79.6. Structured Data Classification#
In addition to the above computer vision and natural
language processing, Auto-Keras can also classify structured
data. The class used for classifying structured data is
StructuredDataClassifier
, and its usage method is roughly the same as that of the
above classes. However, there are some changes in the main
parameters
x
and
y
of the
fit(x,
y)
function of this class.
-
x: Represents the input data for the model. In addition to data types such as
numpy.ndarray
,pandas.DataFrame
, andtensorflow.Dataset
, it can also be a string. This string represents the path to the csv file where the training data is located. In other words, we only need to put this file into thefit
function, and we can even skip the step of reading the data. -
y: Represents the output data of the model, i.e., the target. In addition to data types such as
numpy.ndarray
,pandas.DataFrame
, andtensorflow.Dataset
, it can also be a string. This string represents the column name of a certain column in the csv file passed in through x. That is, this column is used as the target, and the other columns are used as input.
Next, let’s use this utility class to complete the classification of the Titanic.
First, let’s load this dataset:
wget -nc "https://cdn.aibydoing.com/aibydoing/files/titanic_eval.csv"
wget -nc "https://cdn.aibydoing.com/aibydoing/files/titanic_train.csv"
--2023-11-14 11:01:33-- https://cdn.aibydoing.com/aibydoing/files/titanic_eval.csv
正在解析主机 cdn.aibydoing.com (cdn.aibydoing.com)... 198.18.7.59
正在连接 cdn.aibydoing.com (cdn.aibydoing.com)|198.18.7.59|:443... 已连接。
已发出 HTTP 请求,正在等待回应... 200 OK
长度:13049 (13K) [text/csv]
正在保存至: “titanic_eval.csv”
titanic_eval.csv 100%[===================>] 12.74K --.-KB/s 用时 0.08s
2023-11-14 11:01:34 (152 KB/s) - 已保存 “titanic_eval.csv” [13049/13049])
--2023-11-14 11:01:34-- https://cdn.aibydoing.com/aibydoing/files/titanic_train.csv
正在解析主机 cdn.aibydoing.com (cdn.aibydoing.com)... 198.18.7.59
正在连接 cdn.aibydoing.com (cdn.aibydoing.com)|198.18.7.59|:443... 已连接。
已发出 HTTP 请求,正在等待回应... 200 OK
长度:30874 (30K) [text/csv]
正在保存至: “titanic_train.csv”
titanic_train.csv 100%[===================>] 30.15K --.-KB/s 用时 0.1s
2023-11-14 11:01:36 (258 KB/s) - 已保存 “titanic_train.csv” [30874/30874])
import pandas as pd
df = pd.read_csv("titanic_train.csv")
df.head()
survived | sex | age | n_siblings_spouses | parch | fare | class | deck | embark_town | alone | |
---|---|---|---|---|---|---|---|---|---|---|
0 | 0 | male | 22.0 | 1 | 0 | 7.2500 | Third | unknown | Southampton | n |
1 | 1 | female | 38.0 | 1 | 0 | 71.2833 | First | C | Cherbourg | n |
2 | 1 | female | 26.0 | 0 | 0 | 7.9250 | Third | unknown | Southampton | y |
3 | 1 | female | 35.0 | 1 | 0 | 53.1000 | First | C | Southampton | n |
4 | 0 | male | 28.0 | 0 | 0 | 8.4583 | Third | unknown | Queenstown | y |
From the above results, we can see that the table stores some basic information of the passengers on the Titanic, and “survived” in it indicates whether the passenger survived the accident. The purpose of this experiment is to train through Auto-Keras to obtain a classification model that can predict whether a passenger was rescued (where 1 represents being rescued and 0 represents not being rescued).
We don’t need to perform any processing on the above data. We can directly put the data into the classifier for classification as follows. (The following code may take 8 - 10 minutes to run. Please be patient and wait.)
import autokeras as ak
# 定义分类器,这里我们尝试 3 个模型,并最终返回三个中的最佳模型
clf = ak.StructuredDataClassifier(max_trials=3)
# 只需传入文件路径和文件列名即可
# verbose=2 表示每一个 epoch 显示一次日志信息
clf.fit(x='titanic_train.csv', y='survived', verbose=2, epochs=500)
print("训练完成")
Trial 3 Complete [00h 00m 04s]
val_accuracy: 0.8695651888847351
Best val_accuracy So Far: 0.8782608509063721
Total elapsed time: 00h 00m 24s
Epoch 1/500
20/20 - 0s - loss: 0.6435 - accuracy: 0.6970 - 236ms/epoch - 12ms/step
Epoch 2/500
20/20 - 0s - loss: 0.5628 - accuracy: 0.7974 - 13ms/epoch - 632us/step
Epoch 3/500
20/20 - 0s - loss: 0.5063 - accuracy: 0.8086 - 13ms/epoch - 634us/step
Epoch 4/500
20/20 - 0s - loss: 0.4680 - accuracy: 0.8166 - 15ms/epoch - 744us/step
Epoch 5/500
20/20 - 0s - loss: 0.4453 - accuracy: 0.8198 - 12ms/epoch - 605us/step
Epoch 6/500
20/20 - 0s - loss: 0.4316 - accuracy: 0.8214 - 12ms/epoch - 593us/step
Epoch 7/500
20/20 - 0s - loss: 0.4226 - accuracy: 0.8246 - 16ms/epoch - 777us/step
Epoch 8/500
20/20 - 0s - loss: 0.4156 - accuracy: 0.8230 - 18ms/epoch - 904us/step
Epoch 9/500
20/20 - 0s - loss: 0.4097 - accuracy: 0.8278 - 12ms/epoch - 613us/step
Epoch 10/500
20/20 - 0s - loss: 0.4051 - accuracy: 0.8309 - 12ms/epoch - 624us/step
Epoch 11/500
20/20 - 0s - loss: 0.4010 - accuracy: 0.8309 - 13ms/epoch - 640us/step
Epoch 12/500
20/20 - 0s - loss: 0.3973 - accuracy: 0.8325 - 18ms/epoch - 891us/step
Epoch 13/500
20/20 - 0s - loss: 0.3941 - accuracy: 0.8341 - 13ms/epoch - 638us/step
Epoch 14/500
20/20 - 0s - loss: 0.3912 - accuracy: 0.8341 - 13ms/epoch - 643us/step
Epoch 15/500
20/20 - 0s - loss: 0.3885 - accuracy: 0.8389 - 12ms/epoch - 582us/step
Epoch 16/500
20/20 - 0s - loss: 0.3862 - accuracy: 0.8373 - 12ms/epoch - 581us/step
Epoch 17/500
20/20 - 0s - loss: 0.3838 - accuracy: 0.8373 - 12ms/epoch - 616us/step
Epoch 18/500
20/20 - 0s - loss: 0.3818 - accuracy: 0.8421 - 14ms/epoch - 689us/step
Epoch 19/500
20/20 - 0s - loss: 0.3797 - accuracy: 0.8453 - 12ms/epoch - 608us/step
Epoch 20/500
20/20 - 0s - loss: 0.3779 - accuracy: 0.8437 - 11ms/epoch - 572us/step
Epoch 21/500
20/20 - 0s - loss: 0.3762 - accuracy: 0.8437 - 11ms/epoch - 573us/step
Epoch 22/500
20/20 - 0s - loss: 0.3746 - accuracy: 0.8453 - 13ms/epoch - 657us/step
Epoch 23/500
20/20 - 0s - loss: 0.3730 - accuracy: 0.8469 - 13ms/epoch - 626us/step
Epoch 24/500
20/20 - 0s - loss: 0.3716 - accuracy: 0.8453 - 12ms/epoch - 595us/step
Epoch 25/500
20/20 - 0s - loss: 0.3702 - accuracy: 0.8437 - 13ms/epoch - 642us/step
Epoch 26/500
20/20 - 0s - loss: 0.3688 - accuracy: 0.8437 - 13ms/epoch - 649us/step
Epoch 27/500
20/20 - 0s - loss: 0.3675 - accuracy: 0.8437 - 13ms/epoch - 665us/step
Epoch 28/500
20/20 - 0s - loss: 0.3661 - accuracy: 0.8453 - 12ms/epoch - 620us/step
Epoch 29/500
20/20 - 0s - loss: 0.3649 - accuracy: 0.8453 - 13ms/epoch - 644us/step
Epoch 30/500
20/20 - 0s - loss: 0.3637 - accuracy: 0.8437 - 12ms/epoch - 594us/step
Epoch 31/500
20/20 - 0s - loss: 0.3625 - accuracy: 0.8421 - 11ms/epoch - 571us/step
Epoch 32/500
20/20 - 0s - loss: 0.3613 - accuracy: 0.8421 - 11ms/epoch - 563us/step
Epoch 33/500
20/20 - 0s - loss: 0.3602 - accuracy: 0.8437 - 12ms/epoch - 584us/step
Epoch 34/500
20/20 - 0s - loss: 0.3590 - accuracy: 0.8453 - 11ms/epoch - 553us/step
Epoch 35/500
20/20 - 0s - loss: 0.3580 - accuracy: 0.8453 - 12ms/epoch - 592us/step
Epoch 36/500
20/20 - 0s - loss: 0.3570 - accuracy: 0.8453 - 12ms/epoch - 582us/step
Epoch 37/500
20/20 - 0s - loss: 0.3559 - accuracy: 0.8453 - 11ms/epoch - 572us/step
Epoch 38/500
20/20 - 0s - loss: 0.3550 - accuracy: 0.8437 - 12ms/epoch - 592us/step
Epoch 39/500
20/20 - 0s - loss: 0.3539 - accuracy: 0.8453 - 12ms/epoch - 598us/step
Epoch 40/500
20/20 - 0s - loss: 0.3531 - accuracy: 0.8453 - 13ms/epoch - 638us/step
Epoch 41/500
20/20 - 0s - loss: 0.3521 - accuracy: 0.8469 - 12ms/epoch - 612us/step
Epoch 42/500
20/20 - 0s - loss: 0.3513 - accuracy: 0.8469 - 12ms/epoch - 587us/step
Epoch 43/500
20/20 - 0s - loss: 0.3505 - accuracy: 0.8453 - 13ms/epoch - 636us/step
Epoch 44/500
20/20 - 0s - loss: 0.3495 - accuracy: 0.8453 - 12ms/epoch - 607us/step
Epoch 45/500
20/20 - 0s - loss: 0.3488 - accuracy: 0.8453 - 13ms/epoch - 625us/step
Epoch 46/500
20/20 - 0s - loss: 0.3480 - accuracy: 0.8453 - 12ms/epoch - 617us/step
Epoch 47/500
20/20 - 0s - loss: 0.3472 - accuracy: 0.8453 - 12ms/epoch - 623us/step
Epoch 48/500
20/20 - 0s - loss: 0.3464 - accuracy: 0.8453 - 13ms/epoch - 660us/step
Epoch 49/500
20/20 - 0s - loss: 0.3456 - accuracy: 0.8453 - 12ms/epoch - 601us/step
Epoch 50/500
20/20 - 0s - loss: 0.3449 - accuracy: 0.8453 - 12ms/epoch - 605us/step
Epoch 51/500
20/20 - 0s - loss: 0.3441 - accuracy: 0.8453 - 12ms/epoch - 620us/step
Epoch 52/500
20/20 - 0s - loss: 0.3435 - accuracy: 0.8453 - 12ms/epoch - 606us/step
Epoch 53/500
20/20 - 0s - loss: 0.3425 - accuracy: 0.8437 - 12ms/epoch - 606us/step
Epoch 54/500
20/20 - 0s - loss: 0.3419 - accuracy: 0.8453 - 12ms/epoch - 589us/step
Epoch 55/500
20/20 - 0s - loss: 0.3411 - accuracy: 0.8453 - 11ms/epoch - 561us/step
Epoch 56/500
20/20 - 0s - loss: 0.3403 - accuracy: 0.8501 - 13ms/epoch - 638us/step
Epoch 57/500
20/20 - 0s - loss: 0.3396 - accuracy: 0.8485 - 11ms/epoch - 559us/step
Epoch 58/500
20/20 - 0s - loss: 0.3389 - accuracy: 0.8517 - 11ms/epoch - 561us/step
Epoch 59/500
20/20 - 0s - loss: 0.3382 - accuracy: 0.8501 - 12ms/epoch - 620us/step
Epoch 60/500
20/20 - 0s - loss: 0.3374 - accuracy: 0.8501 - 11ms/epoch - 558us/step
Epoch 61/500
20/20 - 0s - loss: 0.3369 - accuracy: 0.8517 - 11ms/epoch - 558us/step
Epoch 62/500
20/20 - 0s - loss: 0.3359 - accuracy: 0.8517 - 12ms/epoch - 589us/step
Epoch 63/500
20/20 - 0s - loss: 0.3354 - accuracy: 0.8517 - 11ms/epoch - 569us/step
Epoch 64/500
20/20 - 0s - loss: 0.3347 - accuracy: 0.8517 - 12ms/epoch - 586us/step
Epoch 65/500
20/20 - 0s - loss: 0.3339 - accuracy: 0.8533 - 11ms/epoch - 548us/step
Epoch 66/500
20/20 - 0s - loss: 0.3335 - accuracy: 0.8533 - 13ms/epoch - 639us/step
Epoch 67/500
20/20 - 0s - loss: 0.3326 - accuracy: 0.8565 - 13ms/epoch - 646us/step
Epoch 68/500
20/20 - 0s - loss: 0.3321 - accuracy: 0.8533 - 12ms/epoch - 613us/step
Epoch 69/500
20/20 - 0s - loss: 0.3315 - accuracy: 0.8549 - 12ms/epoch - 597us/step
Epoch 70/500
20/20 - 0s - loss: 0.3308 - accuracy: 0.8549 - 12ms/epoch - 623us/step
Epoch 71/500
20/20 - 0s - loss: 0.3302 - accuracy: 0.8533 - 13ms/epoch - 630us/step
Epoch 72/500
20/20 - 0s - loss: 0.3296 - accuracy: 0.8533 - 13ms/epoch - 636us/step
Epoch 73/500
20/20 - 0s - loss: 0.3290 - accuracy: 0.8533 - 14ms/epoch - 683us/step
Epoch 74/500
20/20 - 0s - loss: 0.3285 - accuracy: 0.8549 - 13ms/epoch - 642us/step
Epoch 75/500
20/20 - 0s - loss: 0.3278 - accuracy: 0.8565 - 12ms/epoch - 576us/step
Epoch 76/500
20/20 - 0s - loss: 0.3272 - accuracy: 0.8565 - 12ms/epoch - 597us/step
Epoch 77/500
20/20 - 0s - loss: 0.3268 - accuracy: 0.8581 - 11ms/epoch - 543us/step
Epoch 78/500
20/20 - 0s - loss: 0.3261 - accuracy: 0.8549 - 11ms/epoch - 536us/step
Epoch 79/500
20/20 - 0s - loss: 0.3255 - accuracy: 0.8581 - 12ms/epoch - 614us/step
Epoch 80/500
20/20 - 0s - loss: 0.3249 - accuracy: 0.8565 - 15ms/epoch - 747us/step
Epoch 81/500
20/20 - 0s - loss: 0.3243 - accuracy: 0.8596 - 14ms/epoch - 724us/step
Epoch 82/500
20/20 - 0s - loss: 0.3237 - accuracy: 0.8596 - 12ms/epoch - 593us/step
Epoch 83/500
20/20 - 0s - loss: 0.3231 - accuracy: 0.8612 - 15ms/epoch - 737us/step
Epoch 84/500
20/20 - 0s - loss: 0.3225 - accuracy: 0.8612 - 11ms/epoch - 564us/step
Epoch 85/500
20/20 - 0s - loss: 0.3220 - accuracy: 0.8612 - 12ms/epoch - 621us/step
Epoch 86/500
20/20 - 0s - loss: 0.3215 - accuracy: 0.8612 - 11ms/epoch - 541us/step
Epoch 87/500
20/20 - 0s - loss: 0.3208 - accuracy: 0.8612 - 12ms/epoch - 591us/step
Epoch 88/500
20/20 - 0s - loss: 0.3203 - accuracy: 0.8596 - 11ms/epoch - 553us/step
Epoch 89/500
20/20 - 0s - loss: 0.3198 - accuracy: 0.8596 - 11ms/epoch - 552us/step
Epoch 90/500
20/20 - 0s - loss: 0.3192 - accuracy: 0.8612 - 12ms/epoch - 623us/step
Epoch 91/500
20/20 - 0s - loss: 0.3186 - accuracy: 0.8596 - 12ms/epoch - 606us/step
Epoch 92/500
20/20 - 0s - loss: 0.3180 - accuracy: 0.8612 - 13ms/epoch - 633us/step
Epoch 93/500
20/20 - 0s - loss: 0.3174 - accuracy: 0.8612 - 17ms/epoch - 864us/step
Epoch 94/500
20/20 - 0s - loss: 0.3169 - accuracy: 0.8612 - 44ms/epoch - 2ms/step
Epoch 95/500
20/20 - 0s - loss: 0.3163 - accuracy: 0.8612 - 21ms/epoch - 1ms/step
Epoch 96/500
20/20 - 0s - loss: 0.3158 - accuracy: 0.8628 - 12ms/epoch - 600us/step
Epoch 97/500
20/20 - 0s - loss: 0.3152 - accuracy: 0.8644 - 12ms/epoch - 603us/step
Epoch 98/500
20/20 - 0s - loss: 0.3147 - accuracy: 0.8644 - 30ms/epoch - 1ms/step
Epoch 99/500
20/20 - 0s - loss: 0.3141 - accuracy: 0.8644 - 12ms/epoch - 622us/step
Epoch 100/500
20/20 - 0s - loss: 0.3136 - accuracy: 0.8628 - 12ms/epoch - 590us/step
Epoch 101/500
20/20 - 0s - loss: 0.3131 - accuracy: 0.8644 - 12ms/epoch - 606us/step
Epoch 102/500
20/20 - 0s - loss: 0.3125 - accuracy: 0.8660 - 11ms/epoch - 547us/step
Epoch 103/500
20/20 - 0s - loss: 0.3119 - accuracy: 0.8644 - 11ms/epoch - 559us/step
Epoch 104/500
20/20 - 0s - loss: 0.3114 - accuracy: 0.8644 - 11ms/epoch - 560us/step
Epoch 105/500
20/20 - 0s - loss: 0.3109 - accuracy: 0.8628 - 11ms/epoch - 555us/step
Epoch 106/500
20/20 - 0s - loss: 0.3104 - accuracy: 0.8644 - 11ms/epoch - 551us/step
Epoch 107/500
20/20 - 0s - loss: 0.3098 - accuracy: 0.8644 - 11ms/epoch - 554us/step
Epoch 108/500
20/20 - 0s - loss: 0.3092 - accuracy: 0.8628 - 11ms/epoch - 563us/step
Epoch 109/500
20/20 - 0s - loss: 0.3088 - accuracy: 0.8660 - 11ms/epoch - 554us/step
Epoch 110/500
20/20 - 0s - loss: 0.3083 - accuracy: 0.8660 - 11ms/epoch - 567us/step
Epoch 111/500
20/20 - 0s - loss: 0.3076 - accuracy: 0.8644 - 11ms/epoch - 553us/step
Epoch 112/500
20/20 - 0s - loss: 0.3073 - accuracy: 0.8676 - 11ms/epoch - 553us/step
Epoch 113/500
20/20 - 0s - loss: 0.3067 - accuracy: 0.8676 - 11ms/epoch - 547us/step
Epoch 114/500
20/20 - 0s - loss: 0.3062 - accuracy: 0.8676 - 11ms/epoch - 556us/step
Epoch 115/500
20/20 - 0s - loss: 0.3056 - accuracy: 0.8676 - 12ms/epoch - 594us/step
Epoch 116/500
20/20 - 0s - loss: 0.3051 - accuracy: 0.8676 - 13ms/epoch - 645us/step
Epoch 117/500
20/20 - 0s - loss: 0.3046 - accuracy: 0.8660 - 12ms/epoch - 586us/step
Epoch 118/500
20/20 - 0s - loss: 0.3042 - accuracy: 0.8692 - 12ms/epoch - 594us/step
Epoch 119/500
20/20 - 0s - loss: 0.3036 - accuracy: 0.8676 - 12ms/epoch - 578us/step
Epoch 120/500
20/20 - 0s - loss: 0.3031 - accuracy: 0.8724 - 12ms/epoch - 579us/step
Epoch 121/500
20/20 - 0s - loss: 0.3025 - accuracy: 0.8724 - 12ms/epoch - 590us/step
Epoch 122/500
20/20 - 0s - loss: 0.3022 - accuracy: 0.8724 - 12ms/epoch - 588us/step
Epoch 123/500
20/20 - 0s - loss: 0.3016 - accuracy: 0.8724 - 12ms/epoch - 586us/step
Epoch 124/500
20/20 - 0s - loss: 0.3012 - accuracy: 0.8708 - 12ms/epoch - 586us/step
Epoch 125/500
20/20 - 0s - loss: 0.3005 - accuracy: 0.8740 - 12ms/epoch - 586us/step
Epoch 126/500
20/20 - 0s - loss: 0.3002 - accuracy: 0.8740 - 12ms/epoch - 585us/step
Epoch 127/500
20/20 - 0s - loss: 0.2996 - accuracy: 0.8740 - 12ms/epoch - 583us/step
Epoch 128/500
20/20 - 0s - loss: 0.2991 - accuracy: 0.8724 - 11ms/epoch - 573us/step
Epoch 129/500
20/20 - 0s - loss: 0.2987 - accuracy: 0.8724 - 12ms/epoch - 584us/step
Epoch 130/500
20/20 - 0s - loss: 0.2983 - accuracy: 0.8724 - 12ms/epoch - 585us/step
Epoch 131/500
20/20 - 0s - loss: 0.2977 - accuracy: 0.8740 - 11ms/epoch - 574us/step
Epoch 132/500
20/20 - 0s - loss: 0.2973 - accuracy: 0.8740 - 12ms/epoch - 592us/step
Epoch 133/500
20/20 - 0s - loss: 0.2967 - accuracy: 0.8756 - 12ms/epoch - 587us/step
Epoch 134/500
20/20 - 0s - loss: 0.2964 - accuracy: 0.8740 - 11ms/epoch - 564us/step
Epoch 135/500
20/20 - 0s - loss: 0.2959 - accuracy: 0.8756 - 11ms/epoch - 572us/step
Epoch 136/500
20/20 - 0s - loss: 0.2954 - accuracy: 0.8740 - 12ms/epoch - 578us/step
Epoch 137/500
20/20 - 0s - loss: 0.2950 - accuracy: 0.8740 - 12ms/epoch - 601us/step
Epoch 138/500
20/20 - 0s - loss: 0.2944 - accuracy: 0.8756 - 11ms/epoch - 562us/step
Epoch 139/500
20/20 - 0s - loss: 0.2941 - accuracy: 0.8740 - 12ms/epoch - 611us/step
Epoch 140/500
20/20 - 0s - loss: 0.2935 - accuracy: 0.8740 - 11ms/epoch - 557us/step
Epoch 141/500
20/20 - 0s - loss: 0.2931 - accuracy: 0.8740 - 12ms/epoch - 596us/step
Epoch 142/500
20/20 - 0s - loss: 0.2926 - accuracy: 0.8740 - 12ms/epoch - 580us/step
Epoch 143/500
20/20 - 0s - loss: 0.2922 - accuracy: 0.8756 - 11ms/epoch - 573us/step
Epoch 144/500
20/20 - 0s - loss: 0.2917 - accuracy: 0.8756 - 12ms/epoch - 586us/step
Epoch 145/500
20/20 - 0s - loss: 0.2912 - accuracy: 0.8740 - 11ms/epoch - 569us/step
Epoch 146/500
20/20 - 0s - loss: 0.2910 - accuracy: 0.8740 - 11ms/epoch - 574us/step
Epoch 147/500
20/20 - 0s - loss: 0.2903 - accuracy: 0.8740 - 11ms/epoch - 569us/step
Epoch 148/500
20/20 - 0s - loss: 0.2900 - accuracy: 0.8756 - 12ms/epoch - 605us/step
Epoch 149/500
20/20 - 0s - loss: 0.2896 - accuracy: 0.8740 - 11ms/epoch - 560us/step
Epoch 150/500
20/20 - 0s - loss: 0.2891 - accuracy: 0.8756 - 12ms/epoch - 577us/step
Epoch 151/500
20/20 - 0s - loss: 0.2886 - accuracy: 0.8772 - 12ms/epoch - 598us/step
Epoch 152/500
20/20 - 0s - loss: 0.2882 - accuracy: 0.8788 - 12ms/epoch - 583us/step
Epoch 153/500
20/20 - 0s - loss: 0.2878 - accuracy: 0.8772 - 12ms/epoch - 594us/step
Epoch 154/500
20/20 - 0s - loss: 0.2874 - accuracy: 0.8788 - 12ms/epoch - 579us/step
Epoch 155/500
20/20 - 0s - loss: 0.2869 - accuracy: 0.8756 - 12ms/epoch - 579us/step
Epoch 156/500
20/20 - 0s - loss: 0.2866 - accuracy: 0.8788 - 11ms/epoch - 564us/step
Epoch 157/500
20/20 - 0s - loss: 0.2861 - accuracy: 0.8756 - 11ms/epoch - 572us/step
Epoch 158/500
20/20 - 0s - loss: 0.2854 - accuracy: 0.8772 - 11ms/epoch - 565us/step
Epoch 159/500
20/20 - 0s - loss: 0.2853 - accuracy: 0.8772 - 11ms/epoch - 564us/step
Epoch 160/500
20/20 - 0s - loss: 0.2847 - accuracy: 0.8772 - 11ms/epoch - 570us/step
Epoch 161/500
20/20 - 0s - loss: 0.2843 - accuracy: 0.8772 - 11ms/epoch - 566us/step
Epoch 162/500
20/20 - 0s - loss: 0.2840 - accuracy: 0.8788 - 12ms/epoch - 587us/step
Epoch 163/500
20/20 - 0s - loss: 0.2834 - accuracy: 0.8788 - 11ms/epoch - 574us/step
Epoch 164/500
20/20 - 0s - loss: 0.2831 - accuracy: 0.8788 - 12ms/epoch - 606us/step
Epoch 165/500
20/20 - 0s - loss: 0.2827 - accuracy: 0.8788 - 11ms/epoch - 561us/step
Epoch 166/500
20/20 - 0s - loss: 0.2821 - accuracy: 0.8788 - 12ms/epoch - 589us/step
Epoch 167/500
20/20 - 0s - loss: 0.2819 - accuracy: 0.8788 - 11ms/epoch - 570us/step
Epoch 168/500
20/20 - 0s - loss: 0.2812 - accuracy: 0.8804 - 11ms/epoch - 568us/step
Epoch 169/500
20/20 - 0s - loss: 0.2811 - accuracy: 0.8804 - 12ms/epoch - 580us/step
Epoch 170/500
20/20 - 0s - loss: 0.2805 - accuracy: 0.8804 - 12ms/epoch - 587us/step
Epoch 171/500
20/20 - 0s - loss: 0.2802 - accuracy: 0.8804 - 11ms/epoch - 570us/step
Epoch 172/500
20/20 - 0s - loss: 0.2798 - accuracy: 0.8804 - 13ms/epoch - 657us/step
Epoch 173/500
20/20 - 0s - loss: 0.2793 - accuracy: 0.8804 - 12ms/epoch - 588us/step
Epoch 174/500
20/20 - 0s - loss: 0.2789 - accuracy: 0.8804 - 12ms/epoch - 578us/step
Epoch 175/500
20/20 - 0s - loss: 0.2786 - accuracy: 0.8804 - 12ms/epoch - 593us/step
Epoch 176/500
20/20 - 0s - loss: 0.2779 - accuracy: 0.8804 - 12ms/epoch - 595us/step
Epoch 177/500
20/20 - 0s - loss: 0.2779 - accuracy: 0.8788 - 12ms/epoch - 580us/step
Epoch 178/500
20/20 - 0s - loss: 0.2773 - accuracy: 0.8804 - 12ms/epoch - 587us/step
Epoch 179/500
20/20 - 0s - loss: 0.2769 - accuracy: 0.8804 - 12ms/epoch - 598us/step
Epoch 180/500
20/20 - 0s - loss: 0.2765 - accuracy: 0.8804 - 12ms/epoch - 583us/step
Epoch 181/500
20/20 - 0s - loss: 0.2760 - accuracy: 0.8804 - 12ms/epoch - 586us/step
Epoch 182/500
20/20 - 0s - loss: 0.2757 - accuracy: 0.8804 - 12ms/epoch - 582us/step
Epoch 183/500
20/20 - 0s - loss: 0.2753 - accuracy: 0.8804 - 12ms/epoch - 596us/step
Epoch 184/500
20/20 - 0s - loss: 0.2748 - accuracy: 0.8804 - 12ms/epoch - 590us/step
Epoch 185/500
20/20 - 0s - loss: 0.2745 - accuracy: 0.8804 - 12ms/epoch - 596us/step
Epoch 186/500
20/20 - 0s - loss: 0.2741 - accuracy: 0.8804 - 12ms/epoch - 583us/step
Epoch 187/500
20/20 - 0s - loss: 0.2737 - accuracy: 0.8804 - 12ms/epoch - 583us/step
Epoch 188/500
20/20 - 0s - loss: 0.2734 - accuracy: 0.8804 - 12ms/epoch - 585us/step
Epoch 189/500
20/20 - 0s - loss: 0.2729 - accuracy: 0.8804 - 12ms/epoch - 589us/step
Epoch 190/500
20/20 - 0s - loss: 0.2727 - accuracy: 0.8852 - 12ms/epoch - 588us/step
Epoch 191/500
20/20 - 0s - loss: 0.2719 - accuracy: 0.8820 - 12ms/epoch - 584us/step
Epoch 192/500
20/20 - 0s - loss: 0.2717 - accuracy: 0.8804 - 12ms/epoch - 598us/step
Epoch 193/500
20/20 - 0s - loss: 0.2714 - accuracy: 0.8852 - 12ms/epoch - 588us/step
Epoch 194/500
20/20 - 0s - loss: 0.2709 - accuracy: 0.8836 - 11ms/epoch - 551us/step
Epoch 195/500
20/20 - 0s - loss: 0.2705 - accuracy: 0.8852 - 12ms/epoch - 603us/step
Epoch 196/500
20/20 - 0s - loss: 0.2702 - accuracy: 0.8836 - 12ms/epoch - 593us/step
Epoch 197/500
20/20 - 0s - loss: 0.2698 - accuracy: 0.8868 - 12ms/epoch - 578us/step
Epoch 198/500
20/20 - 0s - loss: 0.2694 - accuracy: 0.8900 - 12ms/epoch - 599us/step
Epoch 199/500
20/20 - 0s - loss: 0.2689 - accuracy: 0.8900 - 12ms/epoch - 587us/step
Epoch 200/500
20/20 - 0s - loss: 0.2687 - accuracy: 0.8900 - 12ms/epoch - 584us/step
Epoch 201/500
20/20 - 0s - loss: 0.2682 - accuracy: 0.8931 - 12ms/epoch - 599us/step
Epoch 202/500
20/20 - 0s - loss: 0.2678 - accuracy: 0.8931 - 11ms/epoch - 552us/step
Epoch 203/500
20/20 - 0s - loss: 0.2676 - accuracy: 0.8931 - 12ms/epoch - 586us/step
Epoch 204/500
20/20 - 0s - loss: 0.2672 - accuracy: 0.8931 - 12ms/epoch - 599us/step
Epoch 205/500
20/20 - 0s - loss: 0.2668 - accuracy: 0.8931 - 12ms/epoch - 582us/step
Epoch 206/500
20/20 - 0s - loss: 0.2664 - accuracy: 0.8931 - 12ms/epoch - 585us/step
Epoch 207/500
20/20 - 0s - loss: 0.2661 - accuracy: 0.8931 - 12ms/epoch - 583us/step
Epoch 208/500
20/20 - 0s - loss: 0.2658 - accuracy: 0.8947 - 12ms/epoch - 592us/step
Epoch 209/500
20/20 - 0s - loss: 0.2653 - accuracy: 0.8931 - 12ms/epoch - 579us/step
Epoch 210/500
20/20 - 0s - loss: 0.2651 - accuracy: 0.8963 - 11ms/epoch - 574us/step
Epoch 211/500
20/20 - 0s - loss: 0.2647 - accuracy: 0.8931 - 22ms/epoch - 1ms/step
Epoch 212/500
20/20 - 0s - loss: 0.2644 - accuracy: 0.8931 - 12ms/epoch - 579us/step
Epoch 213/500
20/20 - 0s - loss: 0.2639 - accuracy: 0.8947 - 12ms/epoch - 593us/step
Epoch 214/500
20/20 - 0s - loss: 0.2636 - accuracy: 0.8963 - 12ms/epoch - 593us/step
Epoch 215/500
20/20 - 0s - loss: 0.2634 - accuracy: 0.8947 - 12ms/epoch - 622us/step
Epoch 216/500
20/20 - 0s - loss: 0.2629 - accuracy: 0.8963 - 12ms/epoch - 588us/step
Epoch 217/500
20/20 - 0s - loss: 0.2626 - accuracy: 0.8947 - 12ms/epoch - 584us/step
Epoch 218/500
20/20 - 0s - loss: 0.2622 - accuracy: 0.8963 - 12ms/epoch - 585us/step
Epoch 219/500
20/20 - 0s - loss: 0.2619 - accuracy: 0.8963 - 12ms/epoch - 606us/step
Epoch 220/500
20/20 - 0s - loss: 0.2614 - accuracy: 0.8963 - 12ms/epoch - 587us/step
Epoch 221/500
20/20 - 0s - loss: 0.2614 - accuracy: 0.8979 - 12ms/epoch - 598us/step
Epoch 222/500
20/20 - 0s - loss: 0.2609 - accuracy: 0.8979 - 12ms/epoch - 592us/step
Epoch 223/500
20/20 - 0s - loss: 0.2604 - accuracy: 0.8963 - 12ms/epoch - 603us/step
Epoch 224/500
20/20 - 0s - loss: 0.2603 - accuracy: 0.8979 - 11ms/epoch - 572us/step
Epoch 225/500
20/20 - 0s - loss: 0.2597 - accuracy: 0.8979 - 12ms/epoch - 585us/step
Epoch 226/500
20/20 - 0s - loss: 0.2597 - accuracy: 0.8979 - 12ms/epoch - 597us/step
Epoch 227/500
20/20 - 0s - loss: 0.2591 - accuracy: 0.8979 - 11ms/epoch - 548us/step
Epoch 228/500
20/20 - 0s - loss: 0.2589 - accuracy: 0.8979 - 12ms/epoch - 605us/step
Epoch 229/500
20/20 - 0s - loss: 0.2587 - accuracy: 0.8979 - 11ms/epoch - 537us/step
Epoch 230/500
20/20 - 0s - loss: 0.2582 - accuracy: 0.8979 - 12ms/epoch - 596us/step
Epoch 231/500
20/20 - 0s - loss: 0.2580 - accuracy: 0.8979 - 12ms/epoch - 576us/step
Epoch 232/500
20/20 - 0s - loss: 0.2575 - accuracy: 0.8979 - 12ms/epoch - 604us/step
Epoch 233/500
20/20 - 0s - loss: 0.2572 - accuracy: 0.8979 - 12ms/epoch - 575us/step
Epoch 234/500
20/20 - 0s - loss: 0.2571 - accuracy: 0.8979 - 13ms/epoch - 651us/step
Epoch 235/500
20/20 - 0s - loss: 0.2566 - accuracy: 0.8979 - 12ms/epoch - 607us/step
Epoch 236/500
20/20 - 0s - loss: 0.2564 - accuracy: 0.8979 - 12ms/epoch - 591us/step
Epoch 237/500
20/20 - 0s - loss: 0.2561 - accuracy: 0.8995 - 12ms/epoch - 596us/step
Epoch 238/500
20/20 - 0s - loss: 0.2556 - accuracy: 0.8995 - 12ms/epoch - 601us/step
Epoch 239/500
20/20 - 0s - loss: 0.2556 - accuracy: 0.8995 - 12ms/epoch - 598us/step
Epoch 240/500
20/20 - 0s - loss: 0.2551 - accuracy: 0.8995 - 12ms/epoch - 583us/step
Epoch 241/500
20/20 - 0s - loss: 0.2549 - accuracy: 0.8995 - 12ms/epoch - 582us/step
Epoch 242/500
20/20 - 0s - loss: 0.2544 - accuracy: 0.8995 - 12ms/epoch - 594us/step
Epoch 243/500
20/20 - 0s - loss: 0.2542 - accuracy: 0.8995 - 12ms/epoch - 585us/step
Epoch 244/500
20/20 - 0s - loss: 0.2538 - accuracy: 0.9027 - 12ms/epoch - 593us/step
Epoch 245/500
20/20 - 0s - loss: 0.2538 - accuracy: 0.9011 - 12ms/epoch - 595us/step
Epoch 246/500
20/20 - 0s - loss: 0.2533 - accuracy: 0.9027 - 12ms/epoch - 583us/step
Epoch 247/500
20/20 - 0s - loss: 0.2531 - accuracy: 0.9027 - 12ms/epoch - 608us/step
Epoch 248/500
20/20 - 0s - loss: 0.2526 - accuracy: 0.9027 - 12ms/epoch - 575us/step
Epoch 249/500
20/20 - 0s - loss: 0.2525 - accuracy: 0.9027 - 12ms/epoch - 579us/step
Epoch 250/500
20/20 - 0s - loss: 0.2521 - accuracy: 0.9027 - 12ms/epoch - 587us/step
Epoch 251/500
20/20 - 0s - loss: 0.2516 - accuracy: 0.9027 - 12ms/epoch - 596us/step
Epoch 252/500
20/20 - 0s - loss: 0.2516 - accuracy: 0.9011 - 12ms/epoch - 586us/step
Epoch 253/500
20/20 - 0s - loss: 0.2515 - accuracy: 0.9027 - 12ms/epoch - 598us/step
Epoch 254/500
20/20 - 0s - loss: 0.2507 - accuracy: 0.9059 - 12ms/epoch - 599us/step
Epoch 255/500
20/20 - 0s - loss: 0.2509 - accuracy: 0.9043 - 12ms/epoch - 580us/step
Epoch 256/500
20/20 - 0s - loss: 0.2502 - accuracy: 0.9043 - 12ms/epoch - 587us/step
Epoch 257/500
20/20 - 0s - loss: 0.2501 - accuracy: 0.9043 - 12ms/epoch - 585us/step
Epoch 258/500
20/20 - 0s - loss: 0.2498 - accuracy: 0.9027 - 12ms/epoch - 611us/step
Epoch 259/500
20/20 - 0s - loss: 0.2494 - accuracy: 0.9027 - 12ms/epoch - 605us/step
Epoch 260/500
20/20 - 0s - loss: 0.2491 - accuracy: 0.9075 - 12ms/epoch - 587us/step
Epoch 261/500
20/20 - 0s - loss: 0.2488 - accuracy: 0.9075 - 12ms/epoch - 589us/step
Epoch 262/500
20/20 - 0s - loss: 0.2486 - accuracy: 0.9059 - 12ms/epoch - 586us/step
Epoch 263/500
20/20 - 0s - loss: 0.2482 - accuracy: 0.9075 - 12ms/epoch - 591us/step
Epoch 264/500
20/20 - 0s - loss: 0.2477 - accuracy: 0.9059 - 12ms/epoch - 603us/step
Epoch 265/500
20/20 - 0s - loss: 0.2481 - accuracy: 0.9043 - 12ms/epoch - 609us/step
Epoch 266/500
20/20 - 0s - loss: 0.2472 - accuracy: 0.9091 - 12ms/epoch - 601us/step
Epoch 267/500
20/20 - 0s - loss: 0.2473 - accuracy: 0.9091 - 12ms/epoch - 578us/step
Epoch 268/500
20/20 - 0s - loss: 0.2470 - accuracy: 0.9075 - 12ms/epoch - 601us/step
Epoch 269/500
20/20 - 0s - loss: 0.2465 - accuracy: 0.9091 - 12ms/epoch - 617us/step
Epoch 270/500
20/20 - 0s - loss: 0.2465 - accuracy: 0.9091 - 12ms/epoch - 608us/step
Epoch 271/500
20/20 - 0s - loss: 0.2460 - accuracy: 0.9091 - 12ms/epoch - 610us/step
Epoch 272/500
20/20 - 0s - loss: 0.2458 - accuracy: 0.9075 - 13ms/epoch - 641us/step
Epoch 273/500
20/20 - 0s - loss: 0.2455 - accuracy: 0.9075 - 12ms/epoch - 604us/step
Epoch 274/500
20/20 - 0s - loss: 0.2453 - accuracy: 0.9091 - 12ms/epoch - 612us/step
Epoch 275/500
20/20 - 0s - loss: 0.2450 - accuracy: 0.9107 - 12ms/epoch - 597us/step
Epoch 276/500
20/20 - 0s - loss: 0.2447 - accuracy: 0.9107 - 13ms/epoch - 639us/step
Epoch 277/500
20/20 - 0s - loss: 0.2444 - accuracy: 0.9123 - 12ms/epoch - 608us/step
Epoch 278/500
20/20 - 0s - loss: 0.2444 - accuracy: 0.9107 - 13ms/epoch - 639us/step
Epoch 279/500
20/20 - 0s - loss: 0.2439 - accuracy: 0.9091 - 12ms/epoch - 580us/step
Epoch 280/500
20/20 - 0s - loss: 0.2439 - accuracy: 0.9091 - 11ms/epoch - 559us/step
Epoch 281/500
20/20 - 0s - loss: 0.2434 - accuracy: 0.9107 - 11ms/epoch - 549us/step
Epoch 282/500
20/20 - 0s - loss: 0.2434 - accuracy: 0.9107 - 11ms/epoch - 556us/step
Epoch 283/500
20/20 - 0s - loss: 0.2429 - accuracy: 0.9123 - 11ms/epoch - 556us/step
Epoch 284/500
20/20 - 0s - loss: 0.2427 - accuracy: 0.9091 - 11ms/epoch - 555us/step
Epoch 285/500
20/20 - 0s - loss: 0.2424 - accuracy: 0.9107 - 11ms/epoch - 557us/step
Epoch 286/500
20/20 - 0s - loss: 0.2423 - accuracy: 0.9107 - 11ms/epoch - 567us/step
Epoch 287/500
20/20 - 0s - loss: 0.2419 - accuracy: 0.9107 - 11ms/epoch - 560us/step
Epoch 288/500
20/20 - 0s - loss: 0.2416 - accuracy: 0.9107 - 11ms/epoch - 547us/step
Epoch 289/500
20/20 - 0s - loss: 0.2414 - accuracy: 0.9107 - 11ms/epoch - 546us/step
Epoch 290/500
20/20 - 0s - loss: 0.2414 - accuracy: 0.9123 - 11ms/epoch - 541us/step
Epoch 291/500
20/20 - 0s - loss: 0.2408 - accuracy: 0.9107 - 11ms/epoch - 557us/step
Epoch 292/500
20/20 - 0s - loss: 0.2408 - accuracy: 0.9123 - 11ms/epoch - 552us/step
Epoch 293/500
20/20 - 0s - loss: 0.2402 - accuracy: 0.9107 - 11ms/epoch - 538us/step
Epoch 294/500
20/20 - 0s - loss: 0.2402 - accuracy: 0.9107 - 11ms/epoch - 553us/step
Epoch 295/500
20/20 - 0s - loss: 0.2401 - accuracy: 0.9107 - 11ms/epoch - 554us/step
Epoch 296/500
20/20 - 0s - loss: 0.2395 - accuracy: 0.9107 - 11ms/epoch - 545us/step
Epoch 297/500
20/20 - 0s - loss: 0.2394 - accuracy: 0.9123 - 11ms/epoch - 549us/step
Epoch 298/500
20/20 - 0s - loss: 0.2394 - accuracy: 0.9107 - 11ms/epoch - 557us/step
Epoch 299/500
20/20 - 0s - loss: 0.2388 - accuracy: 0.9123 - 11ms/epoch - 552us/step
Epoch 300/500
20/20 - 0s - loss: 0.2387 - accuracy: 0.9107 - 11ms/epoch - 548us/step
Epoch 301/500
20/20 - 0s - loss: 0.2385 - accuracy: 0.9123 - 11ms/epoch - 547us/step
Epoch 302/500
20/20 - 0s - loss: 0.2383 - accuracy: 0.9107 - 11ms/epoch - 548us/step
Epoch 303/500
20/20 - 0s - loss: 0.2380 - accuracy: 0.9123 - 11ms/epoch - 549us/step
Epoch 304/500
20/20 - 0s - loss: 0.2376 - accuracy: 0.9123 - 11ms/epoch - 547us/step
Epoch 305/500
20/20 - 0s - loss: 0.2377 - accuracy: 0.9107 - 11ms/epoch - 546us/step
Epoch 306/500
20/20 - 0s - loss: 0.2373 - accuracy: 0.9107 - 11ms/epoch - 556us/step
Epoch 307/500
20/20 - 0s - loss: 0.2371 - accuracy: 0.9123 - 11ms/epoch - 562us/step
Epoch 308/500
20/20 - 0s - loss: 0.2367 - accuracy: 0.9107 - 11ms/epoch - 568us/step
Epoch 309/500
20/20 - 0s - loss: 0.2367 - accuracy: 0.9107 - 12ms/epoch - 588us/step
Epoch 310/500
20/20 - 0s - loss: 0.2366 - accuracy: 0.9107 - 13ms/epoch - 633us/step
Epoch 311/500
20/20 - 0s - loss: 0.2361 - accuracy: 0.9107 - 12ms/epoch - 591us/step
Epoch 312/500
20/20 - 0s - loss: 0.2360 - accuracy: 0.9107 - 12ms/epoch - 589us/step
Epoch 313/500
20/20 - 0s - loss: 0.2357 - accuracy: 0.9123 - 12ms/epoch - 599us/step
Epoch 314/500
20/20 - 0s - loss: 0.2355 - accuracy: 0.9107 - 11ms/epoch - 572us/step
Epoch 315/500
20/20 - 0s - loss: 0.2351 - accuracy: 0.9107 - 12ms/epoch - 582us/step
Epoch 316/500
20/20 - 0s - loss: 0.2352 - accuracy: 0.9123 - 11ms/epoch - 562us/step
Epoch 317/500
20/20 - 0s - loss: 0.2348 - accuracy: 0.9107 - 12ms/epoch - 587us/step
Epoch 318/500
20/20 - 0s - loss: 0.2346 - accuracy: 0.9107 - 12ms/epoch - 587us/step
Epoch 319/500
20/20 - 0s - loss: 0.2344 - accuracy: 0.9107 - 12ms/epoch - 579us/step
Epoch 320/500
20/20 - 0s - loss: 0.2341 - accuracy: 0.9107 - 12ms/epoch - 599us/step
Epoch 321/500
20/20 - 0s - loss: 0.2341 - accuracy: 0.9107 - 12ms/epoch - 592us/step
Epoch 322/500
20/20 - 0s - loss: 0.2336 - accuracy: 0.9107 - 12ms/epoch - 588us/step
Epoch 323/500
20/20 - 0s - loss: 0.2336 - accuracy: 0.9107 - 12ms/epoch - 599us/step
Epoch 324/500
20/20 - 0s - loss: 0.2331 - accuracy: 0.9123 - 12ms/epoch - 595us/step
Epoch 325/500
20/20 - 0s - loss: 0.2329 - accuracy: 0.9123 - 12ms/epoch - 587us/step
Epoch 326/500
20/20 - 0s - loss: 0.2331 - accuracy: 0.9107 - 19ms/epoch - 974us/step
Epoch 327/500
20/20 - 0s - loss: 0.2326 - accuracy: 0.9107 - 11ms/epoch - 568us/step
Epoch 328/500
20/20 - 0s - loss: 0.2323 - accuracy: 0.9107 - 11ms/epoch - 557us/step
Epoch 329/500
20/20 - 0s - loss: 0.2323 - accuracy: 0.9139 - 11ms/epoch - 552us/step
Epoch 330/500
20/20 - 0s - loss: 0.2320 - accuracy: 0.9091 - 11ms/epoch - 554us/step
Epoch 331/500
20/20 - 0s - loss: 0.2319 - accuracy: 0.9091 - 11ms/epoch - 559us/step
Epoch 332/500
20/20 - 0s - loss: 0.2315 - accuracy: 0.9107 - 11ms/epoch - 561us/step
Epoch 333/500
20/20 - 0s - loss: 0.2312 - accuracy: 0.9107 - 11ms/epoch - 546us/step
Epoch 334/500
20/20 - 0s - loss: 0.2316 - accuracy: 0.9107 - 11ms/epoch - 550us/step
Epoch 335/500
20/20 - 0s - loss: 0.2307 - accuracy: 0.9107 - 12ms/epoch - 611us/step
Epoch 336/500
20/20 - 0s - loss: 0.2310 - accuracy: 0.9091 - 11ms/epoch - 562us/step
Epoch 337/500
20/20 - 0s - loss: 0.2307 - accuracy: 0.9107 - 11ms/epoch - 550us/step
Epoch 338/500
20/20 - 0s - loss: 0.2298 - accuracy: 0.9123 - 12ms/epoch - 576us/step
Epoch 339/500
20/20 - 0s - loss: 0.2308 - accuracy: 0.9107 - 12ms/epoch - 592us/step
Epoch 340/500
20/20 - 0s - loss: 0.2298 - accuracy: 0.9123 - 11ms/epoch - 566us/step
Epoch 341/500
20/20 - 0s - loss: 0.2300 - accuracy: 0.9107 - 12ms/epoch - 592us/step
Epoch 342/500
20/20 - 0s - loss: 0.2297 - accuracy: 0.9107 - 11ms/epoch - 566us/step
Epoch 343/500
20/20 - 0s - loss: 0.2293 - accuracy: 0.9107 - 12ms/epoch - 599us/step
Epoch 344/500
20/20 - 0s - loss: 0.2293 - accuracy: 0.9107 - 11ms/epoch - 569us/step
Epoch 345/500
20/20 - 0s - loss: 0.2290 - accuracy: 0.9107 - 12ms/epoch - 578us/step
Epoch 346/500
20/20 - 0s - loss: 0.2288 - accuracy: 0.9107 - 12ms/epoch - 606us/step
Epoch 347/500
20/20 - 0s - loss: 0.2287 - accuracy: 0.9107 - 12ms/epoch - 577us/step
Epoch 348/500
20/20 - 0s - loss: 0.2286 - accuracy: 0.9107 - 12ms/epoch - 583us/step
Epoch 349/500
20/20 - 0s - loss: 0.2280 - accuracy: 0.9123 - 12ms/epoch - 604us/step
Epoch 350/500
20/20 - 0s - loss: 0.2285 - accuracy: 0.9107 - 12ms/epoch - 594us/step
Epoch 351/500
20/20 - 0s - loss: 0.2276 - accuracy: 0.9123 - 11ms/epoch - 575us/step
Epoch 352/500
20/20 - 0s - loss: 0.2277 - accuracy: 0.9123 - 12ms/epoch - 581us/step
Epoch 353/500
20/20 - 0s - loss: 0.2275 - accuracy: 0.9139 - 11ms/epoch - 560us/step
Epoch 354/500
20/20 - 0s - loss: 0.2273 - accuracy: 0.9139 - 12ms/epoch - 577us/step
Epoch 355/500
20/20 - 0s - loss: 0.2273 - accuracy: 0.9139 - 11ms/epoch - 573us/step
Epoch 356/500
20/20 - 0s - loss: 0.2268 - accuracy: 0.9155 - 11ms/epoch - 574us/step
Epoch 357/500
20/20 - 0s - loss: 0.2267 - accuracy: 0.9139 - 12ms/epoch - 595us/step
Epoch 358/500
20/20 - 0s - loss: 0.2266 - accuracy: 0.9123 - 12ms/epoch - 579us/step
Epoch 359/500
20/20 - 0s - loss: 0.2267 - accuracy: 0.9123 - 12ms/epoch - 586us/step
Epoch 360/500
20/20 - 0s - loss: 0.2260 - accuracy: 0.9139 - 11ms/epoch - 574us/step
Epoch 361/500
20/20 - 0s - loss: 0.2262 - accuracy: 0.9123 - 11ms/epoch - 572us/step
Epoch 362/500
20/20 - 0s - loss: 0.2257 - accuracy: 0.9139 - 11ms/epoch - 562us/step
Epoch 363/500
20/20 - 0s - loss: 0.2258 - accuracy: 0.9139 - 12ms/epoch - 609us/step
Epoch 364/500
20/20 - 0s - loss: 0.2252 - accuracy: 0.9139 - 12ms/epoch - 583us/step
Epoch 365/500
20/20 - 0s - loss: 0.2255 - accuracy: 0.9123 - 12ms/epoch - 587us/step
Epoch 366/500
20/20 - 0s - loss: 0.2249 - accuracy: 0.9123 - 12ms/epoch - 582us/step
Epoch 367/500
20/20 - 0s - loss: 0.2250 - accuracy: 0.9139 - 12ms/epoch - 588us/step
Epoch 368/500
20/20 - 0s - loss: 0.2247 - accuracy: 0.9139 - 12ms/epoch - 586us/step
Epoch 369/500
20/20 - 0s - loss: 0.2245 - accuracy: 0.9139 - 12ms/epoch - 587us/step
Epoch 370/500
20/20 - 0s - loss: 0.2245 - accuracy: 0.9155 - 12ms/epoch - 586us/step
Epoch 371/500
20/20 - 0s - loss: 0.2241 - accuracy: 0.9155 - 12ms/epoch - 594us/step
Epoch 372/500
20/20 - 0s - loss: 0.2240 - accuracy: 0.9139 - 11ms/epoch - 550us/step
Epoch 373/500
20/20 - 0s - loss: 0.2238 - accuracy: 0.9123 - 12ms/epoch - 600us/step
Epoch 374/500
20/20 - 0s - loss: 0.2237 - accuracy: 0.9139 - 12ms/epoch - 578us/step
Epoch 375/500
20/20 - 0s - loss: 0.2235 - accuracy: 0.9139 - 12ms/epoch - 601us/step
Epoch 376/500
20/20 - 0s - loss: 0.2229 - accuracy: 0.9139 - 11ms/epoch - 571us/step
Epoch 377/500
20/20 - 0s - loss: 0.2233 - accuracy: 0.9139 - 12ms/epoch - 585us/step
Epoch 378/500
20/20 - 0s - loss: 0.2227 - accuracy: 0.9155 - 11ms/epoch - 539us/step
Epoch 379/500
20/20 - 0s - loss: 0.2226 - accuracy: 0.9139 - 12ms/epoch - 614us/step
Epoch 380/500
20/20 - 0s - loss: 0.2227 - accuracy: 0.9155 - 12ms/epoch - 612us/step
Epoch 381/500
20/20 - 0s - loss: 0.2222 - accuracy: 0.9139 - 12ms/epoch - 607us/step
Epoch 382/500
20/20 - 0s - loss: 0.2221 - accuracy: 0.9139 - 12ms/epoch - 591us/step
Epoch 383/500
20/20 - 0s - loss: 0.2221 - accuracy: 0.9155 - 12ms/epoch - 582us/step
Epoch 384/500
20/20 - 0s - loss: 0.2219 - accuracy: 0.9155 - 12ms/epoch - 590us/step
Epoch 385/500
20/20 - 0s - loss: 0.2217 - accuracy: 0.9171 - 12ms/epoch - 598us/step
Epoch 386/500
20/20 - 0s - loss: 0.2215 - accuracy: 0.9155 - 12ms/epoch - 599us/step
Epoch 387/500
20/20 - 0s - loss: 0.2212 - accuracy: 0.9139 - 12ms/epoch - 586us/step
Epoch 388/500
20/20 - 0s - loss: 0.2210 - accuracy: 0.9155 - 12ms/epoch - 600us/step
Epoch 389/500
20/20 - 0s - loss: 0.2211 - accuracy: 0.9171 - 12ms/epoch - 618us/step
Epoch 390/500
20/20 - 0s - loss: 0.2204 - accuracy: 0.9139 - 13ms/epoch - 658us/step
Epoch 391/500
20/20 - 0s - loss: 0.2210 - accuracy: 0.9155 - 25ms/epoch - 1ms/step
Epoch 392/500
20/20 - 0s - loss: 0.2203 - accuracy: 0.9155 - 35ms/epoch - 2ms/step
Epoch 393/500
20/20 - 0s - loss: 0.2204 - accuracy: 0.9155 - 17ms/epoch - 848us/step
Epoch 394/500
20/20 - 0s - loss: 0.2199 - accuracy: 0.9171 - 20ms/epoch - 1ms/step
Epoch 395/500
20/20 - 0s - loss: 0.2202 - accuracy: 0.9171 - 18ms/epoch - 879us/step
Epoch 396/500
20/20 - 0s - loss: 0.2198 - accuracy: 0.9155 - 14ms/epoch - 716us/step
Epoch 397/500
20/20 - 0s - loss: 0.2194 - accuracy: 0.9155 - 11ms/epoch - 561us/step
Epoch 398/500
20/20 - 0s - loss: 0.2198 - accuracy: 0.9155 - 11ms/epoch - 544us/step
Epoch 399/500
20/20 - 0s - loss: 0.2194 - accuracy: 0.9155 - 11ms/epoch - 550us/step
Epoch 400/500
20/20 - 0s - loss: 0.2190 - accuracy: 0.9171 - 11ms/epoch - 561us/step
Epoch 401/500
20/20 - 0s - loss: 0.2191 - accuracy: 0.9155 - 11ms/epoch - 549us/step
Epoch 402/500
20/20 - 0s - loss: 0.2187 - accuracy: 0.9139 - 11ms/epoch - 541us/step
Epoch 403/500
20/20 - 0s - loss: 0.2187 - accuracy: 0.9155 - 11ms/epoch - 562us/step
Epoch 404/500
20/20 - 0s - loss: 0.2185 - accuracy: 0.9155 - 11ms/epoch - 544us/step
Epoch 405/500
20/20 - 0s - loss: 0.2186 - accuracy: 0.9155 - 11ms/epoch - 548us/step
Epoch 406/500
20/20 - 0s - loss: 0.2181 - accuracy: 0.9155 - 11ms/epoch - 544us/step
Epoch 407/500
20/20 - 0s - loss: 0.2177 - accuracy: 0.9155 - 12ms/epoch - 594us/step
Epoch 408/500
20/20 - 0s - loss: 0.2183 - accuracy: 0.9171 - 11ms/epoch - 570us/step
Epoch 409/500
20/20 - 0s - loss: 0.2174 - accuracy: 0.9155 - 11ms/epoch - 564us/step
Epoch 410/500
20/20 - 0s - loss: 0.2179 - accuracy: 0.9171 - 11ms/epoch - 549us/step
Epoch 411/500
20/20 - 0s - loss: 0.2173 - accuracy: 0.9171 - 11ms/epoch - 562us/step
Epoch 412/500
20/20 - 0s - loss: 0.2170 - accuracy: 0.9155 - 11ms/epoch - 564us/step
Epoch 413/500
20/20 - 0s - loss: 0.2172 - accuracy: 0.9171 - 11ms/epoch - 553us/step
Epoch 414/500
20/20 - 0s - loss: 0.2168 - accuracy: 0.9171 - 11ms/epoch - 549us/step
Epoch 415/500
20/20 - 0s - loss: 0.2167 - accuracy: 0.9171 - 12ms/epoch - 582us/step
Epoch 416/500
20/20 - 0s - loss: 0.2168 - accuracy: 0.9171 - 12ms/epoch - 576us/step
Epoch 417/500
20/20 - 0s - loss: 0.2163 - accuracy: 0.9155 - 11ms/epoch - 564us/step
Epoch 418/500
20/20 - 0s - loss: 0.2165 - accuracy: 0.9171 - 11ms/epoch - 565us/step
Epoch 419/500
20/20 - 0s - loss: 0.2160 - accuracy: 0.9171 - 12ms/epoch - 583us/step
Epoch 420/500
20/20 - 0s - loss: 0.2162 - accuracy: 0.9171 - 12ms/epoch - 590us/step
Epoch 421/500
20/20 - 0s - loss: 0.2155 - accuracy: 0.9171 - 11ms/epoch - 568us/step
Epoch 422/500
20/20 - 0s - loss: 0.2160 - accuracy: 0.9171 - 11ms/epoch - 565us/step
Epoch 423/500
20/20 - 0s - loss: 0.2150 - accuracy: 0.9171 - 12ms/epoch - 600us/step
Epoch 424/500
20/20 - 0s - loss: 0.2154 - accuracy: 0.9171 - 12ms/epoch - 592us/step
Epoch 425/500
20/20 - 0s - loss: 0.2153 - accuracy: 0.9171 - 11ms/epoch - 547us/step
Epoch 426/500
20/20 - 0s - loss: 0.2149 - accuracy: 0.9171 - 12ms/epoch - 612us/step
Epoch 427/500
20/20 - 0s - loss: 0.2147 - accuracy: 0.9171 - 11ms/epoch - 564us/step
Epoch 428/500
20/20 - 0s - loss: 0.2150 - accuracy: 0.9171 - 12ms/epoch - 575us/step
Epoch 429/500
20/20 - 0s - loss: 0.2140 - accuracy: 0.9187 - 11ms/epoch - 543us/step
Epoch 430/500
20/20 - 0s - loss: 0.2147 - accuracy: 0.9171 - 12ms/epoch - 575us/step
Epoch 431/500
20/20 - 0s - loss: 0.2137 - accuracy: 0.9171 - 12ms/epoch - 580us/step
Epoch 432/500
20/20 - 0s - loss: 0.2144 - accuracy: 0.9187 - 11ms/epoch - 571us/step
Epoch 433/500
20/20 - 0s - loss: 0.2137 - accuracy: 0.9187 - 12ms/epoch - 586us/step
Epoch 434/500
20/20 - 0s - loss: 0.2138 - accuracy: 0.9187 - 16ms/epoch - 788us/step
Epoch 435/500
20/20 - 0s - loss: 0.2132 - accuracy: 0.9187 - 14ms/epoch - 683us/step
Epoch 436/500
20/20 - 0s - loss: 0.2137 - accuracy: 0.9187 - 13ms/epoch - 638us/step
Epoch 437/500
20/20 - 0s - loss: 0.2132 - accuracy: 0.9187 - 26ms/epoch - 1ms/step
Epoch 438/500
20/20 - 0s - loss: 0.2130 - accuracy: 0.9187 - 15ms/epoch - 736us/step
Epoch 439/500
20/20 - 0s - loss: 0.2129 - accuracy: 0.9171 - 12ms/epoch - 616us/step
Epoch 440/500
20/20 - 0s - loss: 0.2131 - accuracy: 0.9187 - 12ms/epoch - 589us/step
Epoch 441/500
20/20 - 0s - loss: 0.2126 - accuracy: 0.9187 - 11ms/epoch - 555us/step
Epoch 442/500
20/20 - 0s - loss: 0.2126 - accuracy: 0.9187 - 11ms/epoch - 569us/step
Epoch 443/500
20/20 - 0s - loss: 0.2124 - accuracy: 0.9187 - 14ms/epoch - 716us/step
Epoch 444/500
20/20 - 0s - loss: 0.2124 - accuracy: 0.9187 - 12ms/epoch - 586us/step
Epoch 445/500
20/20 - 0s - loss: 0.2121 - accuracy: 0.9203 - 12ms/epoch - 592us/step
Epoch 446/500
20/20 - 0s - loss: 0.2119 - accuracy: 0.9187 - 12ms/epoch - 586us/step
Epoch 447/500
20/20 - 0s - loss: 0.2117 - accuracy: 0.9187 - 12ms/epoch - 584us/step
Epoch 448/500
20/20 - 0s - loss: 0.2117 - accuracy: 0.9187 - 12ms/epoch - 585us/step
Epoch 449/500
20/20 - 0s - loss: 0.2117 - accuracy: 0.9203 - 12ms/epoch - 594us/step
Epoch 450/500
20/20 - 0s - loss: 0.2112 - accuracy: 0.9187 - 12ms/epoch - 575us/step
Epoch 451/500
20/20 - 0s - loss: 0.2113 - accuracy: 0.9187 - 12ms/epoch - 600us/step
Epoch 452/500
20/20 - 0s - loss: 0.2111 - accuracy: 0.9187 - 12ms/epoch - 594us/step
Epoch 453/500
20/20 - 0s - loss: 0.2114 - accuracy: 0.9203 - 12ms/epoch - 598us/step
Epoch 454/500
20/20 - 0s - loss: 0.2106 - accuracy: 0.9203 - 12ms/epoch - 595us/step
Epoch 455/500
20/20 - 0s - loss: 0.2107 - accuracy: 0.9203 - 12ms/epoch - 595us/step
Epoch 456/500
20/20 - 0s - loss: 0.2111 - accuracy: 0.9187 - 12ms/epoch - 609us/step
Epoch 457/500
20/20 - 0s - loss: 0.2105 - accuracy: 0.9187 - 12ms/epoch - 580us/step
Epoch 458/500
20/20 - 0s - loss: 0.2104 - accuracy: 0.9187 - 12ms/epoch - 598us/step
Epoch 459/500
20/20 - 0s - loss: 0.2103 - accuracy: 0.9187 - 12ms/epoch - 612us/step
Epoch 460/500
20/20 - 0s - loss: 0.2100 - accuracy: 0.9187 - 11ms/epoch - 575us/step
Epoch 461/500
20/20 - 0s - loss: 0.2103 - accuracy: 0.9203 - 12ms/epoch - 607us/step
Epoch 462/500
20/20 - 0s - loss: 0.2096 - accuracy: 0.9203 - 12ms/epoch - 596us/step
Epoch 463/500
20/20 - 0s - loss: 0.2101 - accuracy: 0.9187 - 12ms/epoch - 603us/step
Epoch 464/500
20/20 - 0s - loss: 0.2096 - accuracy: 0.9203 - 12ms/epoch - 591us/step
Epoch 465/500
20/20 - 0s - loss: 0.2096 - accuracy: 0.9203 - 12ms/epoch - 595us/step
Epoch 466/500
20/20 - 0s - loss: 0.2093 - accuracy: 0.9187 - 12ms/epoch - 623us/step
Epoch 467/500
20/20 - 0s - loss: 0.2090 - accuracy: 0.9203 - 13ms/epoch - 648us/step
Epoch 468/500
20/20 - 0s - loss: 0.2093 - accuracy: 0.9203 - 13ms/epoch - 675us/step
Epoch 469/500
20/20 - 0s - loss: 0.2091 - accuracy: 0.9203 - 12ms/epoch - 609us/step
Epoch 470/500
20/20 - 0s - loss: 0.2088 - accuracy: 0.9187 - 12ms/epoch - 603us/step
Epoch 471/500
20/20 - 0s - loss: 0.2087 - accuracy: 0.9203 - 12ms/epoch - 613us/step
Epoch 472/500
20/20 - 0s - loss: 0.2087 - accuracy: 0.9219 - 20ms/epoch - 984us/step
Epoch 473/500
20/20 - 0s - loss: 0.2083 - accuracy: 0.9203 - 13ms/epoch - 634us/step
Epoch 474/500
20/20 - 0s - loss: 0.2086 - accuracy: 0.9203 - 12ms/epoch - 622us/step
Epoch 475/500
20/20 - 0s - loss: 0.2084 - accuracy: 0.9187 - 12ms/epoch - 614us/step
Epoch 476/500
20/20 - 0s - loss: 0.2078 - accuracy: 0.9203 - 12ms/epoch - 603us/step
Epoch 477/500
20/20 - 0s - loss: 0.2085 - accuracy: 0.9203 - 12ms/epoch - 613us/step
Epoch 478/500
20/20 - 0s - loss: 0.2074 - accuracy: 0.9203 - 12ms/epoch - 622us/step
Epoch 479/500
20/20 - 0s - loss: 0.2079 - accuracy: 0.9203 - 12ms/epoch - 620us/step
Epoch 480/500
20/20 - 0s - loss: 0.2078 - accuracy: 0.9203 - 13ms/epoch - 626us/step
Epoch 481/500
20/20 - 0s - loss: 0.2073 - accuracy: 0.9203 - 13ms/epoch - 625us/step
Epoch 482/500
20/20 - 0s - loss: 0.2072 - accuracy: 0.9203 - 13ms/epoch - 630us/step
Epoch 483/500
20/20 - 0s - loss: 0.2074 - accuracy: 0.9203 - 11ms/epoch - 563us/step
Epoch 484/500
20/20 - 0s - loss: 0.2070 - accuracy: 0.9203 - 12ms/epoch - 623us/step
Epoch 485/500
20/20 - 0s - loss: 0.2069 - accuracy: 0.9187 - 11ms/epoch - 556us/step
Epoch 486/500
20/20 - 0s - loss: 0.2069 - accuracy: 0.9203 - 11ms/epoch - 556us/step
Epoch 487/500
20/20 - 0s - loss: 0.2069 - accuracy: 0.9203 - 11ms/epoch - 559us/step
Epoch 488/500
20/20 - 0s - loss: 0.2065 - accuracy: 0.9203 - 11ms/epoch - 552us/step
Epoch 489/500
20/20 - 0s - loss: 0.2065 - accuracy: 0.9219 - 11ms/epoch - 558us/step
Epoch 490/500
20/20 - 0s - loss: 0.2064 - accuracy: 0.9203 - 11ms/epoch - 548us/step
Epoch 491/500
20/20 - 0s - loss: 0.2067 - accuracy: 0.9203 - 11ms/epoch - 555us/step
Epoch 492/500
20/20 - 0s - loss: 0.2057 - accuracy: 0.9203 - 11ms/epoch - 540us/step
Epoch 493/500
20/20 - 0s - loss: 0.2066 - accuracy: 0.9219 - 11ms/epoch - 575us/step
Epoch 494/500
20/20 - 0s - loss: 0.2057 - accuracy: 0.9219 - 11ms/epoch - 561us/step
Epoch 495/500
20/20 - 0s - loss: 0.2061 - accuracy: 0.9187 - 11ms/epoch - 547us/step
Epoch 496/500
20/20 - 0s - loss: 0.2057 - accuracy: 0.9219 - 11ms/epoch - 560us/step
Epoch 497/500
20/20 - 0s - loss: 0.2058 - accuracy: 0.9203 - 11ms/epoch - 556us/step
Epoch 498/500
20/20 - 0s - loss: 0.2052 - accuracy: 0.9203 - 11ms/epoch - 546us/step
Epoch 499/500
20/20 - 0s - loss: 0.2057 - accuracy: 0.9219 - 11ms/epoch - 549us/step
Epoch 500/500
20/20 - 0s - loss: 0.2054 - accuracy: 0.9203 - 11ms/epoch - 541us/step
INFO:tensorflow:Assets written to: ./structured_data_classifier/best_model/assets
训练完成
Since the operation speed of structured data is relatively fast, here we tried three models (only one model was tried for the above image and text classification). If you are running locally, you can set the values of max_trials and epochs larger to find a more suitable model.
Next, let me use the best model found to test the test data
in the
titanic_eval.csv
file:
print('Accuracy: {accuracy}'.format(
accuracy=clf.evaluate(x='titanic_eval.csv', y='survived')))
9/9 [==============================] - 0s 708us/step - loss: 0.6394 - accuracy: 0.8182
Accuracy: [0.6393933296203613, 0.8181818127632141]
As can be seen from the results, even when only three models
were tried, the accuracy of the best model is quite good. Of
course, we can try more models to obtain better accuracy.
And in this process, we don’t need complex data processing,
model building and other steps. We only need to pass the
data into the
StructuredDataClassifier
utility class to achieve this. This is the great advantage
of
Auto-Keras
.
79.7. Pre-trained Model#
Currently, Auto-Keras also supports multiple pre-trained model tasks, namely: object detection, sentiment analysis, text classification, speech generation, and speech recognition. You can directly use these pre-trained models to complete rapid inference and prediction. At the same time, you need to install the dependency library autokeras-pretrained that Auto-Keras supports for pre-trained models.
Since these pre-trained models are hosted on Google Cloud,
the download speed in China is extremely slow. The method of
using these pre-trained models is quite simple. The object
detection task for the
example.jpg
image can be completed as follows.
from autokeras_pretrained import ObjectDetector
detector = ObjectDetector()
detector.predict("./example.jpg", output_file_path="./")
For more content, you can refer to the official examples.
79.8. Advantages and Disadvantages Analysis#
The usage of Auto-Keras is very simple. Especially after you
have learned scikit-learn and other deep learning
frameworks, there are basically no obstacles to learning the
use of Auto-Keras. Simply put, in previous modeling tasks,
you needed to import different algorithms from different
modules, then set hyperparameters for the algorithms, and
even needed to use advanced tuning methods such as grid
search. However, in Auto-Keras, you only need to import
ImageClassifier
or
TextClassifier
. If you have enough time, you don’t even need to set
parameters for these two APIs.
But the core problem also emerges, which is time. In the previous examples, in order to obtain results more quickly, the experiment only tried a small number of models. If you try it locally without setting restrictions, the execution time of Auto-Keras is extremely long. The key point is that the above dataset is relatively simple, and the data used in the real environment is often much more complex.
Therefore, through experiments with Auto-Keras, you should be able to further feel the advantages and disadvantages brought by automated deep learning. The advantage lies in its simplicity of use, and you don’t even need to understand the algorithm principle at all. Because it integrates automatic hyperparameter optimization and automatic data processing, it is even much more convenient and faster to use. The disadvantage is that it takes a long time to search, dozens of minutes, several hours or even several days. In addition, automated deep learning is not omnipotent, and it may also happen that it fails to find an ideal model after spending a lot of time. In this case, if handled by a machine learning expert, the problems with the data or algorithm may be discovered more quickly.
Finally, since Auto-Keras is still in its infancy and has many framework issues, it can only be considered barely usable at present. However, the good news is that the team is completely refactoring the Auto-Keras code, and we also look forward to having a better experience in the future.
79.9. Summary#
In this experiment, we learned how to use the Auto-Keras automated deep learning framework. Among them, the methods and steps of applying Auto-Keras to image and text classification problems were mainly introduced, and we became familiar with the API parameters and carried out example exercises. In addition, the focus of the experiment was to experience the advantages and disadvantages brought by automated deep learning, which is very important.
Related Links